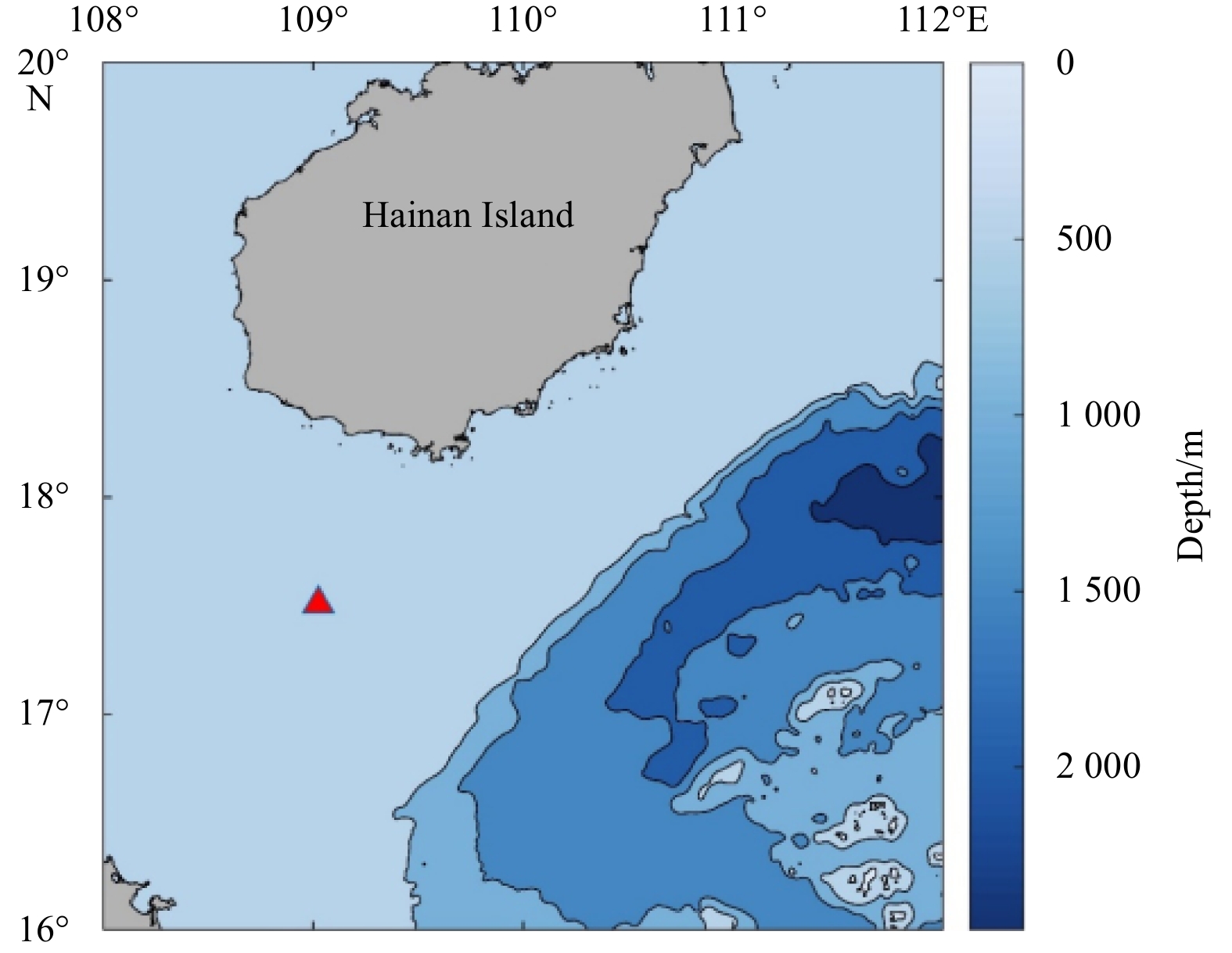
Citation: | Guifen Wang, Wen Zhou, Zhantang Xu, Wenlong Xu, Yuezhong Yang, Wenxi Cao. Vertical variations in the bio-optical properties of seawater in the northern South China Sea during summer 2008[J]. Acta Oceanologica Sinica, 2020, 39(4): 42-56. doi: 10.1007/s13131-020-1535-y |
Internal waves (IWs) are ubiquitous in the ocean and play important roles in energy transfer, biological processes, marine production activities, and ocean engineering. Because IWs frequently occur in the South China Sea (SCS), observing these waves in this region is highly significant (Huang et al., 2016).
In-situ observations, such as acoustic Doppler current profiles and conductivity–temperature–depth, are widely used to observe IWs. These strategies enable the observation of the amplitude, period, phase velocity, polarity, and other characteristics of IWs with high accuracy (Briscoe, 1975) but are usually limited to specific locations. Moreover, they cannot determine the characteristics of IWs at different locations.
Developments in satellite remote sensing have enabled the observation of IWs using optical and microwave satellite images. Synthetic aperture radar (SAR) can obtain high-resolution (several meters) sea surface images, rendering it suitable for observing IWs (Yang et al., 2001); additionally, it has the advantage of being able to conduct all-day, all-weather operations. Alpers (1985) presented a fundamental radar imaging theory of IWs for SAR in 1985, i.e., IW leads to sea-surface convergence or divergence, which changes the surface roughness, thereby affecting the gray level of the radar image. Many characteristics of IWs have been estimated in the literature based on this study, such as the velocity of IWs (Li et al., 2000), the characteristic half-widths of IWs (Zheng et al., 2001), the amplitudes of IWs (Jia et al., 2019), and the spatial distribution of IWs (Liu et al., 1998; Ning et al., 2020). The moderate resolution imaging spectroradiometer (MODIS) has a large swath area of several hundred kilometers, fine spatial resolution, and near-daily global coverage; hence, it is commonly used to study the IWs, in particular, to study characteristics such as the occurrence frequency (Jackson, 2007; Wang et al., 2011), propagation and sources (Sun et al., 2019; Wang et al., 2013), and spatial distribution (Zhou et al., 2016) of IWs.
X-band marine radar has high spatiotemporal resolution and can obtain sea surface images in real-time. Thus, this technology may help determine the evolution of an IW packet (Kropfli et al., 1999). The imaging mechanism of X-band marine radar is similar to that of SAR; specifically, it detects modulations in sea surface roughness associated with currents induced by IWs (Xue et al., 2013). Watson and Robinson (1990) studied the evolution of IWs in the Strait of Gibraltar using X-band marine radar images. Orr and Mignerey (2003) used X-band marine radar to track IWs in the SCS. Ramos et al. (2009) used Radon transform to calculate IW parameters, such as the propagation direction of IWs, their nonlinear velocity, the distance between solitary waves, and the number of solitary waves in each packet, from radar backscatter images. Lü et al. (2010) employed Radon transform to retrieve the propagation direction and velocity of IW packets in the northeastern SCS and then estimated the mixed layer depth by using the two-layer finite depth model. Plant et al. (2011) found at least two different types of IWs in the SCS by analyzing the images of shipborne dual-polarized coherent X-band radar deployed in the SCS. Pan and Jay (2009) used X-band radar images to track IW packets in the Colombia River and subsequently analyzed the characteristics of the detected IWs in combination with the KdV equation. Zha et al. (2012) used X-band radar images in combination with the Morison empirical formula to estimate the force exerted by IWs on a cylindrical pile. Lund et al. (2013) proposed an automated retrieval method of IWs from radar images and discussed the characteristics of IWs with amplitudes greater than 20 m. Badiey et al. (2016) estimated the speed, propagation direction, and amplitude of IWs by analyzing the data obtained from moored thermistor chains and an X-band radar.
Previous studies mostly estimated the average parameters of IWs, such as their average phase velocity and propagation direction (Ramos et al., 2009); unfortunately, these parameters cannot determine the phase velocity of different parts of the waves, which are important for studying the evolution of IWs. However, the distribution of IWs is complex as the latter may show significant changes over areas with a varied topography. Thus, obtaining the small-scale evolution of IWs using traditional methods is challenging. This study proposes a novel method to extract the phase velocity vector of each point on the crest of IWs using X-band marine radar images. The rest of this paper is organized as follows. Section 2 introduces the experimental data, Section 3 presents the method of extracting IW parameters from X-band radar images, and Section 4 verifies and discusses the method. Finally, the conclusions are presented in Section 5.
An experiment was performed in September 2017 on an oil platform in the SCS. The observed sea area lies ~100 km south of Hainan Island (Fig. 1), where the IWs appear frequently (Liang et al., 2019; Jia et al., 2021). The water depth of the region is ~90 m, and the water depth gradually decreases from southeast to northwest. The tides in the observed sea area are mainly irregular diurnal tides. IWs often appear and propagate from southeast to northwest or from east to west (Wang et al., 2013). The X-band marine radar used in the experiment is developed based on a commercial Furuno Far-2127 shipborne navigation radar. Data acquisition software is developed to convert the backscatter from the sea surface into an 8-bit gray value image (Chen et al., 2019). The main configurations of the radar are listed in Table 1. The antenna rotation speed is 42 r/min, and hence, the temporal resolution of the radar is 1.43 s. A single radar image sequence contains 32–128 radar images, and the time difference between two adjacent image sequences is 3–10 min. The radial resolution of the radar is 3.75 m, and the measurement ranges were ~6 km. As an example, Fig. 2 shows two images collected by the X-band radar when IWs pass; here, the wide adjacent bright and dark bands reflect radar echoes caused by changes in sea surface roughness due to IWs, and small wind waves are superimposed on the wide bands. The wave crests of the IW packet are regular in Fig. 2a but intersect in Fig. 2b. Thus, the variation of IWs in this area is complex.
Parameter | Value |
Polarization | HH |
Radar frequency/MHz | 9 410±30 |
Pulse width/ns | 70 |
Pulse repetition frequency/Hz | 3 000 |
Transmit power/kW | 25 |
Beamwidth (3 dB)/(°) | ~1 horizontally, ~20 vertically |
Antenna | 2.4 m slotted waveguide antenna |
Antenna rotation speed/(r·min−1) | 42 |
Sample frequency/MHz | 40 |
Given the limitations of the experimental conditions, no in-situ measurement of IWs was conducted. Instead, synchronous MODIS images of the region were selected to verify the IWs recorded by the X-band marine radar images. The MODIS sensors are onboard the National Aeronautics and Space Administration’s Earth Observing System satellites Terra and Aqua (Jackson, 2007). Terra and aqua transit at 10:30 (local time) and 13:30 (local time) every day. The MODIS has a swath width of 2 330 km and a spatial resolution of 250 m; it can be used to observe ocean IWs at a large scale. Because the optical satellite images are easily blocked by clouds, only two groups of matched X-band marine radar images and MODIS images were obtained.
This section describes the method of extracting IW parameters from X-band marine radar images, including the phase velocities on the crests of the IWs, the number of solitary waves, and their distances in the IW packet.
The radar image sequence is first averaged to reduce the influence of wind waves on the X-band marine radar images. For an X-band marine radar image sequence,
Two adjacent radar image sequences must be selected, i.e.,
The backscatter intensity of X-band radar decreases with increasing distance (Lund et al., 2013). Thus, removing the ramp of gray values with distance is necessary to extract the characteristics of IWs effectively.
The power function is used to fit the gray values of the averaged radar image for each
$$ {\bar I_{{\rm{f}}i}}\left( {r,{\varphi _0},t} \right) = a \cdot {r^b}\quad \left( {i = 1,2} \right) , $$ | (1) |
where a and b are coefficients that can be determined using the least-squares method.
The ramp of the radar images is then corrected as follows:
$$ {\bar I_{{\rm{c}}i}}\left( {r,{\varphi _0},t} \right) = {\bar I_i}\left( {r,{\varphi _0},t} \right) - {\bar I_{{\rm{f}}i}}\left( {r,{\varphi _0},t} \right)\quad \left( {i = 1,2} \right) . $$ | (2) |
Following radar backscatter ramp correction, the gray values of the radar image are adjusted to the range of 0–255 as follows:
$$ {\bar I_{{\rm{c}}i}}\left( {r,\varphi ,t} \right) = \frac{{{{\bar I}_{{\rm{c}}i}}\left( {r,\varphi ,t} \right) - \min \left( {{{\bar I}_{{\rm{c}}i}}\left( {r,\varphi ,t} \right)} \right)}}{{\max \left( {{{\bar I}_{{\rm{c}}i}}\left( {r,\varphi ,t} \right)} \right) - \min \left( {{{\bar I}_{{\rm{c}}i}}\left( {r,\varphi ,t} \right)} \right)}} \times 255 . $$ | (3) |
Two-dimensional fast Fourier transform (2D-FFT) is applied to the radar images to obtain the average propagation direction of the IWs. The 2D wavenumber spectrum of the IWs is then obtained. The average propagation direction of the IW packet can be obtained using
$$ {\varphi _{\rm{p}}} = \arctan \left( {\frac{{{k_{y\max }}}}{{{k_{x\max }}}}} \right) , $$ | (4) |
where the wavenumbers
According to Eq. (4), the propagation direction of an IW has an ambiguity of 180°. Thus, cross-spectral analysis of the two adjacent radar images is used to determine the actual propagation direction of IWs (Chen et al., 2019).
The radar radial profiles at
$$ {v_i} = \frac{{\Delta {r_i}}}{{\Delta t}}\quad \left( {i = 1,2, \ldots } \right) , $$ | (5) |
where
Owing to the small observation range of X-band marine radar, a radar image may not cover all ISWs of an IW packet. Thus, the number of ISWs and the average phase velocity of the IW packet may have to be determined using multiple X-band radar image sequences.
The optical flow method is widely used in moving-target tracking and dynamically analyzes images according to the characteristics of each pixel (Horn and Schunck, 1981). It is used herein to track IWs and extract the phase velocity vector on different locations of the IW. In this method, the gray value of the image before and after motion remains unchanged as a prerequisite,
$$ \frac{{{\rm d} \bar I}}{{{\rm d} t}} = 0 . $$ | (6) |
Using the chain rule for differentiation, we find that the equation of optical flow for a gray image observed by X-band marine radar is
$$ {\bar I_x}u + {\bar I_y}v + {\bar I_t} = 0 , $$ | (7) |
where
$$ {E_{rr}}^2 = \iint {\left[ {{{\left( {{I_x}u + {I_y}v + {I_t}} \right)}^2} + \text{λ} \left( {u_x^2 + u_y^2 + v_x^2 + v_y^2} \right)} \right]{\rm d}x{\rm d}y} , $$ | (8) |
which is usually replaced by the following equation in practice,
$$ {E_{rr}}^2 = \iint {\left[ {{{\left( {{I_x}u + {I_y}v + {I_t}} \right)}^2} + \text{λ} \left( {{{\left( {u - \bar u} \right)}^2} + {{\left( {v - \bar v} \right)}^2}} \right)} \right]{\rm d}x{\rm d}y} , $$ | (9) |
where λ is an unknown weighting factor because the value of it needs to consider the noise in the X-band radar image. Solving this extreme problem, we can determine the velocity of each point in the image as follows:
$$ \left\{\begin{array}{l}{u^{n + 1}} = {{\bar u}^n} - {{\bar I}_x}\dfrac{{{{\bar I}_x}{{\bar u}^n} + {{\bar I}_y}{{\bar v}^n} + {{\bar I}_t}}}{{{\text{λ} ^2} + \bar I_x^2 + \bar I_y^2}},\\ {v^{n + 1}} = {{\bar v}^n} - {{\bar I}_y}\dfrac{{{{\bar I}_x}{{\bar u}^n} + {{\bar I}_y}{{\bar v}^n} + {{\bar I}_t}}}{{{\text{λ} ^2} + \bar I_x^2 + \bar I_y^2}}.\\ \end{array}\right. $$ | (10) |
The average phase velocity (Eq. (5)) estimated from the radial profiles at
First, the two adjacent radar images
Second, the phase velocity of each ISW in the wave packet is estimated using the method described in Section 3.2, and the average phase velocity of the IW is used as the reference phase velocity. For convenience, each peak in the radial profile at
Third, the weighting factor is set to change from
The method to estimate IW parameters from X-band marine radar images is shown in Fig. 3.
Different types of IW images observed by X-band radar (i.e., a set of single IW packet and a set of crossed ISWs) are used to estimate the IW parameters and verify the proposed method for retrieving IW parameters from X-band radar images. Because in-situ measurements are not conducted in the experiment, the MODIS images are used to verify the distribution of IWs. The phase velocities estimated by the proposed optical flow-based method are verified using the average phase velocity of the reference point.
A MODIS image acquired on September 2, 2017, at 03:30 UTC is shown in Fig. 4; in the figure, multiple groups of IW packets may be observed. A group of IW packets located approximately 9.1 km to the west of the radar station could be observed to propagate from east to west. According to the empirical formula in (Tang, 2019), the phase velocity of IWs in the region is approximately 0.76 m/s. Thus, the IW packets would arrive at the radar at ~00:10 UTC on September 1, 2017. The X-band radar image sequence recorded on September 1, 2017, at 23:15 UTC matches the IW in the MODIS image. As shown in Fig. 2a, the propagation direction of the IW packet in the X-band radar image agrees with that determined from the MODIS image (Fig. 4), i.e., the stripes in the X-band marine radar image sequence reflect the IW packet observed by MODIS.
The X-band radar image sequence is averaged using the method described in Section 3.1.1, as shown in Fig. 5. Average processing smoothens the wind waves and swells in the image, thereby facilitating the identification of IW stripes.
The gray values are fitted with the distance determined by the radar by using Eq. (1) to perform ramp correction on the radar image. Figure 6 shows a fitting curve of this group of images. The R-squared value of the fitting results is 0.999 3, which means the selected power function model is applicable to the present case.
The 2D wavenumber spectrum is obtained via 2D FFT of the radar image in the study area (Fig. 7). The propagation direction of the IW in Fig. 7 can be calculated as 90° or 270° (Eq. (4)), and the actual propagation direction of the IW packet can be determined. Cross-spectral analysis reveals that the IW packet propagates from east to west, as shown by the red dashed line in Fig. 7.
After determining the propagation direction of the IW, the radial profiles of the two adjacent radar image sequences are selected at the azimuth of this direction, as shown in Fig. 8. The IW packet contains four ISWs, the distances between which are 219 m, 158 m, and 227 m. The phase velocities at each reference point of the IW packet are 1.01 m/s (leading wave), 1.09 m/s, 1.14 m/s, and 1.18 m/s (Eq. (5)). Typically, the leading wave in an IW packet shows the maximum amplitude; thus, the backscatter intensity caused by the leading wave is largest in the radar image while those caused by the latter ISWs gradually decrease, consistent with the changes in the gray values in Fig. 8.
Histogram equalization is then applied to the two average adjacent radar images (Fig. 9a) to reduce the attenuation of gray values with the range. The images are converted into binary images by selecting a threshold gray value (Fig. 9b), and regions with less than 50 pixels are removed from the binary images (Fig. 9c) to enhance the extraction of IW information.
The value of
Radial profiles | Optical flow-based method | X-band radar (Orr and Mignerey, 2003) | SAR observations (Liang et al., 2019) | Empirical formula (Tang, 2019) | |
Reference point A2 | 1.09 m/s | 1.11 m/s | 1.17 m/s | 0.89 m/s | 0.76 m/s |
Reference point A3 | 1.14 m/s | 1.06 m/s | 1.17 m/s | 0.89 m/s | 0.76 m/s |
Reference point A4 | 1.18 m/s | 1.11 m/s | 1.17 m/s | 0.89 m/s | 0.76 m/s |
The MODIS image obtained on September 11, 2017, at 03:24 UTC is shown in Fig. 11. Two groups of IW packets may be observed at ~13 km to the east and southeast of the radar station, and these packets propagate from east to west and from southeast to northwest, respectively. According to the empirical formula proposed by Tang (2019), the IW packet will arrive at the radar station at ~08:09 UTC on September 11, 2017. The matched X-band radar image sequence was recorded on September 11, 2017, at 07:12 UTC, as shown in Fig. 2b, and the propagation directions of the two IW packets detected agree with those shown in the MODIS image (Fig. 11). In other words, X-band marine radar correctly observed the two groups of IW packets.
The X-band radar image sequences are first preprocessed, after which 2D-FFT is used to obtain the IW wavenumber spectrum, which shows two main spectral peaks. The propagation directions of the two ISWs, which are
The radial profiles
After the radar images are processed via histogram equalization and noise reduction, λ is determined using the phase velocity at reference point B1 to be λ0 = 1.18. The phase velocities of the points on the IW crest are obtained by the optical flow-based method with the selected λ0, as shown in Fig. 14.
Figure 14 shows that the proposed method could detect the propagation directions of the points on the crest of the IWs accurately and that the distribution of phase velocities is reasonable. Some errors in the region of approximately x=0.6 and y=1–2 km may be detected because noise cannot be completely eliminated from the image (Horn and Schunck, 1981). The phase velocity estimated by the optical flow-based method for reference point B2 is 1.15 m/s, which also agrees with the phase velocity of IWs in this region determined in previous studies (Orr and Mignerey, 2003; Liang et al., 2019; Tang, 2019). The phase velocities calculated using different methods are listed in Table 3.
Radial profiles | Optical flow-based method | X-band radar (Orr and Mignerey, 2003) | SAR observations (Liang et al., 2019) | Empirical formula (Tang, 2019) | |
Reference B2 | 1.14 m/s | 1.15 m/s | 1.17 m/s | 0.89 m/s | 0.76 m/s |
Figures 10 and 14 clearly show that the proposed optical flow-based method is applicable not only to single IW packet with parallel ISWs but also to crossed IWs.
In the proposed method, the determination of λ0 is important. To study the sensitivity of the value to the method, the other reference point B2 (Fig. 12), has been chosen to determine the value of λ0. The adjacent two radial profiles along the propagation direction of the ISW B2 are shown in Fig. 15. According to the radial profiles, the average phase velocity at point B2 is estimated to be 1.14 m/s, and the value of λ0 is 1.19. Using the optical flow-based method, the phase velocities of the crossed IWs are estimated, and velocity at the reference point B1 is 0.73 m/s. Compared to the average phase velocity of B1 estimated from the adjacent radial profiles, the relative error of the phase velocity at B1 is 0.01 m/s. Therefore, the proposed method is affected little by the selection of different reference points, i.e., the optical flow-based method is stable.
Phase velocity is one of the most important parameters of IWs. Previous methods of extracting the phase velocity of ISWs mostly estimated the average phase velocity from satellite images. Although the observation range of X-band marine radar is smaller than that of satellite images, X-band marine radar can observe the sea surface in real-time and continuously, which is important for studying the evolution of IWs (Kropfli et al., 1999). Previous methods of extracting the phase velocity of ISWs from X-band radar images were mostly based on Radon transform, which can only estimate the average phase velocity of IWs and cannot obtain the phase velocity of each point on the IWs. A novel method is proposed herein to obtain the phase velocity vector of each point on IWs crest effectively, which is verified using the X-band radar images recorded on an oil platform in the SCS, and the phase velocities estimated by the optical flow-based method are close to those derived from the adjacent radial profiles, as well as the phase velocity of the IWs of the sea area measured by previous studies (Wang et al., 2013; Tang, 2019). Moreover, the proposed method is insensitive to the selection of different reference points; hence, it can reasonably estimate the phase velocities of different parts of IWs. In future research, experiments with in-situ observations of IWs will be performed, and the proposed method will be further evaluated using more data.
[1] |
Barnard A H, Pegau W S, Zaneveld J R V. 1998. Global relationships of the inherent optical properties of the oceans. Journal of Geophysical Research, 103(C11): 24955–24968. doi: 10.1029/98JC01851
|
[2] |
Behrenfeld M J, Boss E. 2003. The beam attenuation to chlorophyll ratio: an optical index of phytoplankton physiology in the surface ocean?. Deep Sea Research Part I: Oceanographic Research Papers, 50(12): 1537–1549. doi: 10.1016/j.dsr.2003.09.002
|
[3] |
Behrenfeld M J, Boss E, Siegel D A, et al. 2005. Carbon-based ocean productivity and phytoplankton physiology from space. Global Biogeochemical Cycles, 19(1): GB1006
|
[4] |
Bishop J K B. 1999. Transmissometer measurement of POC. Deep Sea Research Part I: Oceanographic Research Papers, 46(2): 353–369. doi: 10.1016/S0967-0637(98)00069-7
|
[5] |
Boss E S, Collier R, Larson G, et al. 2007. Measurements of spectral optical properties and their relation to biogeochemical variables and processes in Crater Lake, Crater Lake National Park, OR. Hydrobiologia, 574(1): 149–159. doi: 10.1007/s10750-006-2609-3
|
[6] |
Boss E, Pegau W S, Gardner W D, et al. 2001. Spectral particulate attenuation and particle size distribution in the bottom boundary layer of a continental shelf. Journal of Geophysical Research: Oceans, 106(C5): 9509–9516. doi: 10.1029/2000JC900077
|
[7] |
Boss E, Picheral M, Leeuw T, et al. 2013. The characteristics of particulate absorption, scattering and attenuation coefficients in the surface ocean; Contribution of the Tara Oceans expedition. Methods in Oceanography, 7: 52–62. doi: 10.1016/j.mio.2013.11.002
|
[8] |
Brewin R J W, Dall’Olmo G, Pardo S, et al. 2016. Underway spectrophotometry along the Atlantic Meridional Transect reveals high performance in satellite chlorophyll retrievals. Remote Sensing of Environment, 183: 82–97. doi: 10.1016/j.rse.2016.05.005
|
[9] |
Buesseler K O. 2001. Ocean biogeochemistry and the global carbon cycle: an introduction to the U.S. joint global ocean flux study. Oceanography, 14(4): 5. doi: 10.5670/oceanog.2001.01
|
[10] |
Chang G C, Dickey T D. 2001. Optical and physical variability on timescales from minutes to the seasonal cycle on the New England shelf: July 1996 to June 1997. Journal of Geophysical Research: Oceans, 106(C5): 9435–9453. doi: 10.1029/2000JC900069
|
[11] |
Chen C C, Shiah F K, Chung S W, et al. 2006. Winter phytoplankton blooms in the shallow mixed layer of the South China Sea enhanced by upwelling. Journal of Marine Systems, 59(1–2): 97–110. doi: 10.1016/j.jmarsys.2005.09.002
|
[12] |
Claustre H, Fell F, Oubelkheir K, et al. 2000. Continuous monitoring of surface optical properties across a geostrophic front: Biogeochemical inferences. Limnology and Oceanography, 45(2): 309–321
|
[13] |
Cui Wansong, Wang Difeng, Gong Fang, et al. 2016. The vertical distribution of the beam attenuation coefficient and its correlation to the particulate organic carbon in the north South China Sea. In: Remote Sensing of the Ocean, Sea Ice, Coastal Waters, and Large Water Regions. Edinburgh, United Kingdom: SPIE, 2016
|
[14] |
Cullen J J. 1982. The deep chlorophyll maximum: Comparing vertical profiles of chlorophyll a. Canadian Journal of Fisheries and Aquatic Sciences, 39(5): 791–803. doi: 10.1139/f82-108
|
[15] |
Cullen J J. 2015. Subsurface chlorophyll maximum layers: Enduring enigma or mystery solved?. Annual Review of Marine Science, 7: 207–239. doi: 10.1146/annurev-marine-010213-135111
|
[16] |
Dickey T D. 2001. The role of new technology in advancing ocean biogeochemical research. Oceanography, 14(4): 108–120. doi: 10.5670/oceanog.2001.11
|
[17] |
Fennel K, Boss E. 2003. Subsurface maxima of phytoplankton and chlorophyll: Steady-state solutions from a simple model. Limnology and Oceanography, 48(4): 1521–1534. doi: 10.4319/lo.2003.48.4.1521
|
[18] |
Fujii M, Boss E, Chai F. 2007. The value of adding optics to ecosystem models: a case study. Biogeosciences, 4(5): 817–835. doi: 10.5194/bg-4-817-2007
|
[19] |
Gardner W D, Gundersen J S, Richardson M J, et al. 1999. The role of seasonal and diel changes in mixed-layer depth on carbon and chlorophyll distributions in the Arabian Sea. Deep Sea Research Part II: Topical Studies in Oceanography, 46(8–9): 1833–1858. doi: 10.1016/S0967-0645(99)00046-6
|
[20] |
Gernez P, Antoine D, Huot Y. 2011. Diel cycles of the particulate beam attenuation coefficient under varying trophic conditions in the northwestern Mediterranean Sea: Observations and modeling. Limnology and Oceanography, 56(1): 17–36. doi: 10.4319/lo.2011.56.1.0017
|
[21] |
Gong Xiang, Shi Jie, Gao Huiwang, et al. 2014. Modeling seasonal variations of subsurface chlorophyll maximum in South China Sea. Journal of Ocean University of China, 13(4): 561–571. doi: 10.1007/s11802-014-2060-4
|
[22] |
Gong Xiang, Shi Jinhui, Gao Huiwang, et al. 2015. Steady-state solutions for subsurface chlorophyll maximum in stratified water columns with a bell-shaped vertical profile of chlorophyll. Biogeosciences, 12(4): 905–919. doi: 10.5194/bg-12-905-2015
|
[23] |
Kara A B, Rochford P A, Hurlburt H E. 2000. An optimal definition for ocean mixed layer depth. Journal of Geophysical Research, 105(C7): 16803–16821. doi: 10.1029/2000JC900072
|
[24] |
Kheireddine M, Antoine D. 2014. Diel variability of the beam attenuation and backscattering coefficients in the northwestern Mediterranean Sea (BOUSSOLE site). Journal of Geophysical Research: Oceans, 119(8): 5465–5482. doi: 10.1002/2014JC010007
|
[25] |
Kitchen J C, Zaneveld J R V. 1990. On the noncorrelation of the vertical structure of light scattering and chlorophyll α in case I waters. Journal of Geophysical Research: Oceans, 95(C11): 20237–20246. doi: 10.1029/JC095iC11p20237
|
[26] |
Knap A, Michaels A, Close A, et al. 1996. Protocols for the Joint Global Ocean Flux Study (JGOFS) core measurements. Reprint of the IOC Manuals and Guides No. 29, UNESCO, Paris
|
[27] |
Lavigne H, D’Ortenzio F, D’Alcalà M R, et al. 2015. On the vertical distribution of the chlorophyll a concentration in the Mediterranean Sea: a basin-scale and seasonal approach. Biogeosciences, 12(16): 5021–5039. doi: 10.5194/bg-12-5021-2015
|
[28] |
Li Q P, Dong Y, Wang Y. 2016. Phytoplankton dynamics driven by vertical nutrient fluxes during the spring inter-monsoon period in the northeastern South China Sea. Biogeosciences, 13: 455–466. doi: 10.5194/bg-13-455-2016
|
[29] |
Li Q P, Franks P J S, Landry M R, et al. 2010. Modeling phytoplankton growth rates and chlorophyll to carbon ratios in California coastal and pelagic ecosystems. Journal of Geophysical Research, 115(G4): G04003
|
[30] |
Lin Junfang, Cao Wenxin, Wang Guifen, et al. 2014. Inversion of bio-optical properties in the coastal upwelling waters of the northern South China Sea. Continental Shelf Research, 85: 73–84. doi: 10.1016/j.csr.2014.06.001
|
[31] |
Lin Peigen, Cheng Peng, Gan Jianping, et al. 2016. Dynamics of wind-driven upwelling off the northeastern coast of Hainan Island. Journal of Geophysical Research: Oceans, 121(2): 1160–1173. doi: 10.1002/2015JC011000
|
[32] |
Liu K K, Chao S Y, Shaw P T, et al. 2002. Monsoon-forced chlorophyll distribution and primary production in the South China Sea: observations and a numerical study. Deep Sea Research Part I: Oceanographic Research Papers, 49(8): 1387–1412. doi: 10.1016/S0967-0637(02)00035-3
|
[33] |
Liu Fenfen, Tang Shilin, Chen Chuqun. 2013. Impact of nonlinear mesoscale eddy on phytoplankton distribution in the northern South China Sea. Journal of Marine Systems, 123–124: 33–40. doi: 10.1016/j.jmarsys.2013.04.005
|
[34] |
Lu Zhongming, Gan Jianping, Dai Minhan, et al. 2010. The influence of coastal upwelling and a river plume on the subsurface chlorophyll maximum over the shelf of the northeastern South China Sea. Journal of Marine Systems, 82(1–2): 35–46. doi: 10.1016/j.jmarsys.2010.03.002
|
[35] |
Ma Jinfeng, Zhan Haigang, Du Yan. 2011. Seasonal and interannual variability of surface CDOM in the South China Sea associated with El Niño. Journal of Marine Systems, 85(3–4): 86–95. doi: 10.1016/j.jmarsys.2010.12.006
|
[36] |
McGillicuddy D J Jr. 2016. Mechanisms of physical-biological-biogeochemical interaction at the oceanic mesoscale. Annual Review of Marine Science, 8(1): 125–159. doi: 10.1146/annurev-marine-010814-015606
|
[37] |
McGillicuddy D J Jr, Johnson R, Siegel D A, et al. 1999. Mesoscale variations of biogeochemical properties in the Sargasso Sea. Journal of Geophysical Research: Oceans, 104(C6): 13381–13394. doi: 10.1029/1999JC900021
|
[38] |
Mignot A, Claustre H, D’Ortenzio F, et al. 2011. From the shape of the vertical profile of in vivo fluorescence to Chlorophyll-a concentration. Biogeosciences, 8(8): 2391–2406. doi: 10.5194/bg-8-2391-2011
|
[39] |
Mignot A, Claustre H, Uitz J, et al. 2014. Understanding the seasonal dynamics of phytoplankton biomass and the deep chlorophyll maximum in oligotrophic environments: A Bio-Argo float investigation. Global Biogeochemical Cycles, 28(8): 856–876. doi: 10.1002/2013GB004781
|
[40] |
Mitchell B G, Kahru M, Wieland J, et al. 2003. Determination of spectral absorption coefficients of particles, dissolved material and phytoplankton for discrete water samples. In: Mueller J L, Fargoin G S, McClain C R, eds. Ocean Optics Protocols for Satellite Ocean Color Sensor Validation, revision 4, Vol. IV (Chapter 4), NASA/TM-2003-211621/Rev4-vol. 4. Greenbelt, MD: NASA Goddard Space Flight Center, 39–64
|
[41] |
Mobley C D. 1994. Light and Water: Radiative Transfer in Natural Waters. San Diego, CA, USA: Academic Press
|
[42] |
Morel A. 1988. Optical modeling of the upper ocean in relation to its biogenous matter content (case I waters). Journal of Geophysical Research: Oceans, 93(C9): 10749–10768. doi: 10.1029/JC093iC09p10749
|
[43] |
Morel A, Maritorena S. 2001. Bio-optical properties of oceanic waters: A reappraisal. Journal of Geophysical Research: Oceans, 106(C4): 7163–7180. doi: 10.1029/2000JC000319
|
[44] |
Nencioli F, Chang G, Twardowski M, et al. 2010. Optical characterization of an eddy-induced diatom bloom west of the island of Hawaii. Biogeosciences, 7(1): 151–162. doi: 10.5194/bg-7-151-2010
|
[45] |
Ning Xiuren, Chai Fei, Xue Huijie, et al. 2004. Physical-biological oceanographic couplinginfluencing phytoplankton and primary production in the South China Sea. Journal of Geophysical Research: Oceans, 109: C10005. doi: 10.1029/2004JC002365
|
[46] |
Oubelkheir K, Claustre H, Sciandra A, et al. 2005. Bio-optical and biogeochemical properties of different trophic regimes in oceanic waters. Limnology and Oceanography, 50(6): 1795–1809. doi: 10.4319/lo.2005.50.6.1795
|
[47] |
Oubelkheir K, Sciandra A. 2008. Diel variations in particle stocks in the oligotrophic waters of the Ionian Sea (Mediterranean). Journal of Marine Systems, 74(1–2): 364–371. doi: 10.1016/j.jmarsys.2008.02.008
|
[48] |
Pan Xiaoju, Wong G T F, Tai J H, et al. 2015. Climatology of physical hydrographic and biological characteristics of the Northern South China Sea Shelf-sea (NoSoCS) and adjacent waters: Observations from satellite remote sensing. Deep Sea Research Part II: Topical Studies in Oceanography, 117: 10–22. doi: 10.1016/j.dsr2.2015.02.022
|
[49] |
Parsons T, Takahashi M, Hargrave B. 1984. Biological Oceanographic Processes. 3rd ed. England: Pergamon Press, 330
|
[50] |
Pérez V, Fernández E, Marañón E, et al. 2006. Vertical distribution of phytoplankton biomass, production and growth in the Atlantic subtropical gyres. Deep Sea Research Part I: Oceanographic Research Papers, 53(10): 1616–1634. doi: 10.1016/j.dsr.2006.07.008
|
[51] |
Platt T, Bouman H, Devred E, et al. 2005. Physical forcing and phytoplankton distributions. Scientia Marina, 69(S1): 55–73. doi: 10.3989/scimar.2005.69s155
|
[52] |
Roesler C S, Barnard A H. 2013. Optical proxy for phytoplankton biomass in the absence of photophysiology: Rethinking the absorption line height. Methods in Oceanography, 7: 79–94. doi: 10.1016/j.mio.2013.12.003
|
[53] |
Shang Shaoling, Li Li, Li Jun, et al. 2012. Phytoplankton bloom during the northeast monsoon in the Luzon Strait bordering the Kuroshio. Remote Sensing of Environment, 124: 38–48. doi: 10.1016/j.rse.2012.04.022
|
[54] |
Stramski D, Reynolds R A, Babin M, et al. 2008. Relationships between the surface concentration of particulate organic carbon and optical properties in the eastern South Pacific and eastern Atlantic Oceans. Biogeosciences, 5(1): 171–201. doi: 10.5194/bg-5-171-2008
|
[55] |
Sullivan J M, Twardowski M S, Zaneveld J R V, et al. 2006. Hyperspectral temperature and salt dependencies of absorption by water and heavy water in the 400–750 nm spectral range. Applied Optics, 45(21): 5294–5309. doi: 10.1364/AO.45.005294
|
[56] |
Uitz J, Claustre H, Morel A, et al. 2006. Vertical distribution of phytoplankton communities in open ocean: An assessment based on surface chlorophyll. Journal of Geophysical Research: Oceans, 111(C8): C08005
|
[57] |
Wang Guifen, Cao Wenxi, Yang Dingtian, et al. 2008. Variation in downwelling diffuse attenuation coefficient in the northern South China Sea. Chinese Journal of Oceanology and Limnology, 26(3): 323–333. doi: 10.1007/s00343-008-0323-x
|
[58] |
Wang Lei, Huang Bangqin, Chiang K P, et al. 2016. Physical-biological coupling in the western South China Sea: The response of phytoplankton community to a mesoscale cyclonic eddy. PLoS One, 11(4): e0153735. doi: 10.1371/journal.pone.0153735
|
[59] |
Wang Lei, Huang Bangqin, Laws E A, et al. 2018. Anticyclonic eddy edge effects on phytoplankton communities and particle export in the northern South China Sea. Journal of Geophysical Research: Oceans, 123(11): 7632–7650. doi: 10.1029/2017JC013623
|
[60] |
Wang Dongxiao, Shu Yeqiang, Xue Huijie, et al. 2014. Relative contributions of local wind and topography to the coastal upwelling intensity in the northern South China Sea. Journal of Geophysical Research: Oceans, 119(4): 2550–2567. doi: 10.1002/2013JC009172
|
[61] |
Wang Guifen, Zhou Wen, Cao Wenxi, et al. 2011. Variation of particulate organic carbon and its relationship with bio-optical properties during a phytoplankton bloom in the Pearl River estuary. Marine Pollution Bulletin, 62(9): 1939–1947. doi: 10.1016/j.marpolbul.2011.07.003
|
[62] |
Wong G T F, Pan Xiaoju, Li Kuoyuan, et al. 2015. Hydrography and nutrient dynamics in the Northern South China Sea Shelf-sea (NoSoCS). Deep Sea Research Part II: Topical Studies in Oceanography, 117: 23–40. doi: 10.1016/j.dsr2.2015.02.023
|
[63] |
Xing Xiaogang, Claustre H, Uitz J, et al. 2014. Seasonal variations of bio-optical properties and their interrelationships observed by Bio-Argo floats in the subpolar North Atlantic. Journal of Geophysical Research: Oceans, 119(10): 7372–7388. doi: 10.1002/2014JC010189
|
[64] |
Xiu Peng, Chai Fei. 2014. Connections between physical, optical and biogeochemical processes in the Pacific Ocean. Progress in Oceanography, 122: 30–53. doi: 10.1016/j.pocean.2013.11.008
|
[65] |
Xiu Peng, Liu Yuguang, Li Gang, et al. 2009. Deriving depths of deep chlorophyll maximum and water inherent optical properties: A regional model. Continental Shelf Research, 29(19): 2270–2279. doi: 10.1016/j.csr.2009.09.003
|
[66] |
Xu Wenlong, Wang Guifen, Zhou Wen, et al. 2018. Vertical variability of chlorophyll a concentration and its responses to hydrodynamic processes in the northeastern South China Sea in summer. Journal of Tropical Oceanography (in Chinese), 37(5): 62–73
|
[67] |
Ye H J, Sui Y, Tang D L, et al. 2013. A subsurface chlorophyll a bloom induced by typhoon in the South China Sea. Journal of Marine Systems, 128: 138–145. doi: 10.1016/j.jmarsys.2013.04.010
|
[68] |
Zaneveld J R V, Kitchen J C, Moore C C. 1994. Scattering error correction of reflecting-tube absorption meters. In: Ocean Optics XII. Bergen, Norway: SPIE
|
[69] |
Zhang Wenzhou, Wang Haili, Chai Fei, et al. 2016. Physical drivers of chlorophyll variability in the open South China Sea. Journal of Geophysical Research: Oceans, 121(9): 7123–7140. doi: 10.1002/2016JC011983
|
Parameter | Value |
Polarization | HH |
Radar frequency/MHz | 9 410±30 |
Pulse width/ns | 70 |
Pulse repetition frequency/Hz | 3 000 |
Transmit power/kW | 25 |
Beamwidth (3 dB)/(°) | ~1 horizontally, ~20 vertically |
Antenna | 2.4 m slotted waveguide antenna |
Antenna rotation speed/(r·min−1) | 42 |
Sample frequency/MHz | 40 |
Radial profiles | Optical flow-based method | X-band radar (Orr and Mignerey, 2003) | SAR observations (Liang et al., 2019) | Empirical formula (Tang, 2019) | |
Reference point A2 | 1.09 m/s | 1.11 m/s | 1.17 m/s | 0.89 m/s | 0.76 m/s |
Reference point A3 | 1.14 m/s | 1.06 m/s | 1.17 m/s | 0.89 m/s | 0.76 m/s |
Reference point A4 | 1.18 m/s | 1.11 m/s | 1.17 m/s | 0.89 m/s | 0.76 m/s |
Radial profiles | Optical flow-based method | X-band radar (Orr and Mignerey, 2003) | SAR observations (Liang et al., 2019) | Empirical formula (Tang, 2019) | |
Reference B2 | 1.14 m/s | 1.15 m/s | 1.17 m/s | 0.89 m/s | 0.76 m/s |
Parameter | Value |
Polarization | HH |
Radar frequency/MHz | 9 410±30 |
Pulse width/ns | 70 |
Pulse repetition frequency/Hz | 3 000 |
Transmit power/kW | 25 |
Beamwidth (3 dB)/(°) | ~1 horizontally, ~20 vertically |
Antenna | 2.4 m slotted waveguide antenna |
Antenna rotation speed/(r·min−1) | 42 |
Sample frequency/MHz | 40 |
Radial profiles | Optical flow-based method | X-band radar (Orr and Mignerey, 2003) | SAR observations (Liang et al., 2019) | Empirical formula (Tang, 2019) | |
Reference point A2 | 1.09 m/s | 1.11 m/s | 1.17 m/s | 0.89 m/s | 0.76 m/s |
Reference point A3 | 1.14 m/s | 1.06 m/s | 1.17 m/s | 0.89 m/s | 0.76 m/s |
Reference point A4 | 1.18 m/s | 1.11 m/s | 1.17 m/s | 0.89 m/s | 0.76 m/s |
Radial profiles | Optical flow-based method | X-band radar (Orr and Mignerey, 2003) | SAR observations (Liang et al., 2019) | Empirical formula (Tang, 2019) | |
Reference B2 | 1.14 m/s | 1.15 m/s | 1.17 m/s | 0.89 m/s | 0.76 m/s |