Spatial heterogeneity of copepod community structure and feeding guilds in the nearshore-estuarine waters of Mangalore, southwest coast of India
-
Abstract: The spatial variability of mesozooplankton (MZ) community characteristics, with an emphasis on the predominant taxa, copepods, were evaluated between two distinct coastal water environments of Mangalore: the Netravati-Gurupura estuarine system (NGES) and the adjacent nearshore waters (<20 m depth) on the southwest coast of India during winter 2018. The nearshore waters were characterised by uniformly distributed hydrographic properties, particularly in terms of water column temperature, salinity and turbidity. This well-mixed water column likely stimulated increased phytoplankton chlorophyll a concentrations (av. 2.9±2.2 mg/m3), which in turn supported higher MZ biomass (av. 0.4±0.15 mL/m3) and abundance (av.
6889 ±3387 ind./m3) in the nearshore waters. In contrast, the NGES exhibited highly variable hydrographic conditions, leading to inconsistent chlorophyll a (av. 3.2±3.7 mg/m3), along with significantly lower MZ biomass (av. 0.1±0.2 mL/m3) and abundance (av. 238±339 ind./m3). The MZ community was dominated by herbivorous copepods (HCs), particularly Bestiolina similis, in the entire study region; however, the nearshore waters supported a more diverse taxon. The overall dominance of HCs, e.g., B. similis and Pseudodiaptomous aurivillii, in the nearshore waters, indicates the presence of stable hydrographic conditions, especially consistently higher salinity and chlorophyll a level. In contrast, the unstable hydrographic conditions in the NGES, primarily reflected in the uneven salinity distributions, likely contributed to the reduced MZ biomass and abundance. The relative increase in the abundance of B. similis observed exclusively in the euhaline zones of the estuary highlights the significant influence of neritic waters.-
Key words:
- mesozooplankton /
- Netravati Estuary /
- copepods /
- jellyfish /
- Mangalore.
-
1. Introduction
CO2 is the most important anthropogenic greenhouse gas, and its increasing concentration is accelerating climate change (Liu et al., 2022; IPCC, 2023). Furthermore, water vapor is also an important greenhouse gas (GHG), and changes in its tropospheric concentration affect climate while influencing the concentration of other GHGs in the atmosphere (Wuebbles et al., 1989; Coe et al., 2021). Accurate estimates of air-sea CO2 and water vapor fluxes are crucial to accurately predict and respond to climate change (Hart, 2006; Brevik, 2012; Kaye and Quemada, 2017; Shukla et al., 2019).
In situ observation, using either a box method or a micrometeorological method, is an important method for studying air-sea flux (Hu et al., 2014). For different downwelling environmental conditions and the needs of long-term and continuous in situ observation, the micrometeorological method (based on the principles of atmospheric thermodynamics and dynamics) is the best choice for air-sea flux observation (Siebicke and Emad, 2019). Micrometeorological methods include eddy covariance (EC) methods, mass balance methods, and vorticity accumulation methods (Baldocchi, 2003; Baldocchi et al., 1988; Mammarella et al., 2009; Palatella et al., 2014; Weber, 1999). The EC, which is a direct air-sea flux measurement technique with the advantages of requiring no empirical parameters and being easy to use for in situ observation, has undergone substantial development in recent decades (Horst, 2000; Eugster et al., 2000; Baldocchi, 2003; Shahan et al., 2022).
The eddy correlation method was originally proposed by Swinbank (1951) for the measurement of land-air heat, mass, and momentum changes. With the development of equipment (anemometers, computer storage media) and theory (hydrodynamics, micrometeorology), the method became more widely used (Matthews and Schume, 2022; Shahan et al., 2022; Czubaszek and Wysocka-Czubaszek, 2023; Rytwo and Eliyahou, 2023; Stagakis et al., 2023). As wobble platform calibration methods continue to be refined, there has been an increase in the number of studies focusing on air-sea flux observations carried out on instrumented platforms such as ships, buoys, and offshore platforms (Horst, 2000; Kondo and Tsukamoto, 2007; Miller et al., 2008, 2010; Else et al., 2011; Blomquist et al., 2012).
The rest of this paper is organized as follows. The study area and data, including the experimental platform and instrumentation setup are described in Section 2; the basic principles of the EC, quality control, and quality assessment methods are presented in Section 3; the results of the calibration, quality assessment, and air-sea flux calculations of the high-frequency and low-frequency data are analyzed and discussed in Section 4; finally, conclusions and perspectives are presented in Section 5.
2. Field observation experiment
2.1 Field observation platform
In this study, air-sea CO2 and water vapor flux observation experiments were carried out at the Yantai National Satellite Ocean Calibration Platform (hereafter referred to as the offshore platform) and the Sea Island Observation Platform (hereafter referred to as the sea island) at the Juehua Island Monolithic Beach Pier (Fig. 1). The offshore platform is 20 km away from land, which is conducive to the use of the eddy correlation method for air-sea interface flux observation. The island observation site is between the ocean and the land, which is conducive to understanding offshore air-sea exchange.
In current research on air-sea flux, few scholars have obtained such extensive field observation data for analysis. Many studies often choose to use near-shore meteorological observation stations or coastal scanning LiDAR (sLiDAR) for flux measurements, but both of our two observation platforms are island-based, allowing for direct measurements using instruments. By using a large number of direct sea observation data for subsequent air-sea flux calculations and comparative analysis between high and low frequency instruments, the results promise more accuracy, reliability, and significance for reference.
2.2 Experimental instruments and set-up
The offshore platform was fitted with a LI-
7500 H2O/ CO2 gas analyzer in combination with a Gill Ultrasonic Anemometer HS-100 for air-sea flux observations. Following the installation manual, the LI-7500 was tilted 10–15° during installation to avoid the obstruction of the mirror by water droplets. The 100 Hz CO2 pulsometer (Fig. 2a) and the water vapor pulsometer (Fig. 2b) were developed by the Hefei Institute of Physical Sciences of the Chinese Academy of Sciences, which adopted the TDLAS technology, high-frequency laser modulation technology, high-frequency signal acquisition, and processing technology. An inverse spectral concentration inversion method was used (Li et al., 2021b). The field installation of the high-frequency pulsometer is shown in Fig. 3. The installation needs to avoid shading the ultrasonic anemometer as much as possible to minimize the impact on the wind field.The Licor observation data storage cycle is 4 h. Instrument failure will lead to the loss of the entire 4 h data. High-frequency pulsometer data acquisition and storage can be accomplished at the same time by connecting the instrument to the host computer through the data collector network port for data viewing and export, to facilitate the debugging of the instrument, and prevent data loss.
Only high-frequency observations of water vapor were made on the island. Fig. 3b shows the water vapor field observation platform on Island, which carries an ultrasonic anemometer, a high-frequency water vapor pulsometer, and a Licor analyzer, as shown in Fig. 3d. Instruments were set up at a height of 2 m above the ground at a distance of about 10 m from the shore, which was also necessary to avoid being blocked by surrounding obstacles as much as possible.
2.3 Data
The air-sea flux observations were carried out at the offshore platform and the island in August 2020 and July 2021, respectively. The observations contained three-dimensional wind speed (
$ u $ ,$ v $ ,$ w $ in m/s), water vapor concentration (in 10–6), CO2 concentration (in 10–6), air temperature (in °C), and barometric pressure (in hPa), with the frequency information shown in Table 1 and 2.Table 1. Measuring range of Ultrasonic anemometerInstrument
/parameterWind speed range Wind speed accuracy Wind direction range Wind accuracy Measuring frequency Installation
siteHS-100 0-45 m/s <1.0% RMS 0-359° <±1.0°RMS 100 Hz Island & Platform Table 2. Measuring range of CO2/H2O gas analyzerInstrument/parameter Concentration Measuring frequency Installation site Licor-7500A 0—50 mmol/mol
0—3 000 10–610 Hz Island Licor-7500DS 0—50 mmol/mol
0—3 000 10–620 Hz Platform *CO2/H2O High frequency pulsometer * 100 Hz Island & platform Note: The symbol “*” indicates that the measuring range is limited by reference to the Licor measuring range. Temperature and pressure data were used to facilitate air-sea flux calculations. The units of CO2 and H2O concentrations were converted from volume concentration to bulk density uniformly using the following conversion formula:
$$ \rho =\frac{cPM}{R(T+273.15)} , $$ (1) where
$ \rho $ is the bulk density in mmol·m–3;$ c $ is the volume concentration in 10–6;$ P $ is the pressure in kPa;$ R= 8.314J\cdot{mol}^{-1}\cdot{K}^{-1} $ is the gas constant;$ T $ is the temperature in °C; and$ M $ is the molecular mass in g·mol–1.3. Air-sea flux calculation using the eddy covariance method
3.1 Measurement principle
The eddy covariance method uses fast response sensors to directly measure state variables such as wind speed, temperature, humidity, and carbon dioxide concentration. The covariance of the relevant physical quantities—such as water vapor and carbon dioxide density, with the values of vertically oriented wind speed pulsations (Baldocchi, 2003; Landwehr et al., 2018)—is used to obtain the air-sea fluxes of water vapor and carbon dioxide:
$$ F=\overline{{w}{{\prime}}{\rho }{{\prime}}} , $$ (2) where
$ F $ is the air-sea flux,$ w $ is the vertical wind speed, and$ \rho $ is the water vapor and CO2 volume density; the upper line denotes the pulsation value; and the upper dash denotes the mean value.3.2 Data correction
The first step in air-sea flux calculation using eddy covariance methods involves a series correction of the raw data. Since the high and low-frequency observations are based on the TDLAS and NDIR techniques, respectively, there are some systematic errors in the observations of both. We first performed a systematic error correction on the TDLAS high-frequency pulsometer using the Licor gas analyzer results as a benchmark. The data processing flow after the error correction is shown in Fig. 4. Importantly, the WPL correction compensates for expected physical variability due to temperature and water (Burba, 2013), and the instrumental or measurement errors are not considered. Therefore, only the WPL corrected air-sea fluxes are discussed in Section 4.1.4 of this paper.
3.2.1 Outlier elimination
To minimize the measurement error as much as possible as well as to ensure the integrity of the data information, the measurement noise in this study was preprocessed using the measurement range limitation, outlier rejection of the Pauta criterion, stiff value test, and random pulse rejection. The specific steps are as follows.
(1) Measurement range limitation
According to the measurement range of the instrument, the values beyond the measurement range of the instrument were rejected, as shown in Tables 1 and 2.
(2) Pauta criterion to eliminate outliers
We used the Pauta criterion to identify values outside the range of
$ [\mu -3\delta ,\mu +3\delta ] $ as outliers, where$ \mu $ is the mean and$ \delta $ is the standard deviation.(3) Stiff value test
During the measurement process, the ultrasound probe and the data acquisition system can have consecutive and extremely small changes in data values within a certain period, which is because the difference between neighboring points is less than a certain threshold value. For example, for a time series
$ x $ , the threshold value may be chosen as the width of the probability density function bin of the time series, i.e.,$ \left[\mathrm{m}\mathrm{a}\mathrm{x}\right(x)-\mathrm{m}\mathrm{i}\mathrm{n}(x\left)\right]/100 $ (Vickers and Mahrt, 1997).(4) Random pulse rejection
Random electrical signal pulses in the observation equipment and data acquisition system can lead to random pulses in the data. Random pulse screening was performed as follows: first, a moving window of length 100 was used to calculate its standard deviation; when a data point exceeded the standard deviation by a factor of 3.5 it was regarded as a random pulse and replaced using linear interpolation between data points. Next, the window was moved so that when four or more consecutive points were detected as random pulses, they were not considered as such (Højstrup, 1993; Vickers and Mahrt, 1997).
3.2.2 Detrend
Factors such as changing meteorological conditions and sensor drift can affect data changes. Usually, a systematic increase or decrease in data is considered a linear trend (Rannik and Vesala, 1999). To eliminate such systematic variations, we applied a linear detrending method to the data.
There are
$ N $ samples of data in total, where the data corresponding to the k-th sample point is$ {c}_{k} $ and the corresponding time is$ {t}_{k} $ , the least squares expression is (Lee et al., 2005):$$ {\bar{c}}_{k}=\bar{c}+b\left({t}_{k}-\frac{1}{N}{\sum }_{k=1}^{N}{t}_{k}\right) , $$ (3) where
$ \bar{c} $ refers to the mean value of the sample concentration data,$ {\bar{c}}_{k} $ refers to the value that should be subtracted from$ {c}_{k} $ to remove the trend, which is the sum of$ \bar{c} $ and the trend value at$ {c}_{k} $ , and$ b $ is the slope of the best linearly fitted line for the$ {c}_{k} $ sample:$$ b=\frac{\displaystyle{\sum }_{k=1}^{N}{c}_{k}{t}_{k}-\frac{1}{N}{\sum }_{k=1}^{N}{c}_{k}{\sum }_{k=1}^{N}{t}_{k}}{\displaystyle{\sum }_{k=1}^{N}{t}_{k}{t}_{k}-\frac{1}{N}{\sum }_{k=1}^{N}{t}_{k}{\sum }_{k=1}^{N}{t}_{k}} , $$ (4) The trend value
$ {c}_{{k}_{d}} $ for:$$ {c}_{{k}_{d}}={c}_{k}-{\bar{c}}_{k} . $$ (5) 3.2.3 Delay correction
Open-circuit sensors with a certain processing time delay lead to a certain fixed time delay that causes the overall observed data to be skewed (Mauder and Foken, 2015). The time delay between the ultrasonic anemometer and the gas analyzer is determined by the maximum correlation value between the CO2/H2O concentration and
$ w $ :$$ CC\left(c,w,t\right)=\frac{\displaystyle\frac{1}{N}{\sum }_{i=1}^{N}{c}{\prime}\left(i\right){w}{\prime}\left(i+t\right)}{{\sigma }_{c}{\sigma }_{w}} , $$ (6) $$ {c}{\prime}=c\left(i\right)-\frac{1}{N}{ \displaystyle\text{Σ} }_{j=1}^{N}c\left(j\right) , $$ (7) $$ {w}{\prime}=w\left(i\right)-\frac{1}{N}{ \text{Σ} }_{j=1}^{N}w\left(j\right) , $$ (8) $$ {\sigma }_{c}=\sqrt{\frac{1}{N}{\sum }_{i=1}^{N}{c}{{'}2}} , $$ (9) $$ {\sigma }_{w}=\sqrt{\frac{1}{N}{\sum }_{i=1}^{N}{w}{{'}2}} . $$ (10) where
$ c $ is the CO2/H2O concentration and$ w $ is the vertical wind speed. Variable$ t $ is the time series, generally the length of time before and after 2 s. The time$ {t}_{max} $ when the correlation coefficient is maximized is the delay time between the ultrasonic anemometer and the gas analyzer.3.2.4 Coordinate rotation
The basic condition for the eddy correlation method is that the vertical mean wind speed is zero. For a flat subsurface, the wind speed
$ u $ measured by the ultrasonic anemometer is along the x-axis along the direction of the prevailing wind field in the measurement environment;$ v $ is along the y-axis perpendicular to the x-axis; and$ w $ is along the z-axis perpendicular to the subsurface. Commonly used coordinate rotation methods are the double rotation method (DR), the triple rotation method (TR), and the planar fit method (PF). TR is no longer recommended because it increases wind speed noise and causes greater stress calculation errors (Wilczak et al., 2001). The PF method analyses the total data over the whole period, which is not suitable for the study of this paper at different frequencies of observation.In this paper, the measured wind speed data were corrected using the DR method (Geernaert, 1988; Wilczak et al., 2001).
First, the first rotation is carried out so that
$ \overline{v}=0 $ :$$ \left\{\begin{array}{c}{u}_{1}=u\mathit{cos}\theta +v\text{sin}\theta \\ {v}_{1}=-u\text{sin}\theta +v\text{cos}\theta \\ {w}_{1}=w\end{array}\right. , $$ (11) where
$ \theta ={\mathrm{t}\mathrm{a}\mathrm{n}}^{-1}\left(\dfrac{\bar{v}}{\bar{u}}\right) $ and the subscript “1” indicates the first rotation.Next, a second rotation is carried out so that
$ \bar{w}=0 $ :$$ \left\{\begin{array}{c}{u}_{2}={u}_{1}\text{cos}\phi +{w}_{1}\text{sin}\phi \\ {v}_{2}={v}_{1}\\ {w}_{2}=-{u}_{1}\text{sin}\phi +{w}_{1}\text{cos}\phi \end{array}\right. , $$ (12) where
$ \phi ={\mathrm{t}\mathrm{a}\mathrm{n}}^{-1}\left(\dfrac{\overline{{w}_{1}}}{\overline{{u}_{1}}}\right) $ and the subscript “2” indicates the second rotation.3.2.5 Time match
To compare the effect of different observation frequencies on the air-sea flux, the observations were time-matched. The mean value of the sampled values less than 1/2 of the matched time resolution was the matched point value. The points that were not matched to a value were supplemented with NaN values. Taking the 20 Hz standard time grid as an example, the center moment of its grid is
$ {t}_{m} $ , and the mean value of the measurements$ {x}_{ti} $ at all$ {t}_{i} $ moments that satisfy |$ {t}_{i}-{t}_{m} $ |$ < \dfrac{1}{20}\times \dfrac{1}{2} $ is the matching point value.3.2.6 Webb, Pearman, and Leuning correction
The WPL correction is used to correct for fluctuations in CO2 and H2O densities due to water vapor and temperature fluctuations in open-optical path gas analyzers (Jentzsch et al., 2021).
$$ {F}_{c}=\overline{{w}{\prime}{\rho }_{c}{\prime}}+\mu \frac{\overline{{\rho }_{c}}}{\overline{{\rho }_{a}}}\overline{{w}{\prime}{\rho }_{w}{\prime}}+\left(1+\mu \sigma \right){\bar{\rho }}_{c}\frac{\overline{{w}{\prime}{T}{\prime}}}{\overline{T}} , $$ (13) $$ {F}_{w}=\left(1+\mu \sigma \right)\left(\overline{{w}{\prime}{\rho }_{w}{\prime}}+\overline{{\rho }_{w}}\frac{\overline{{w}{\prime{T}{\prime}}}}{\overline{T}}\right) , $$ (14) where
$ \mu =1.608 $ is the mass ratio of the relative molecules of dry air and water vapor;$ {\rho }_{w} $ is the water vapor volume density,$ {\rho }_{c} $ is the CO2 volume density;$ T $ is the air temperature in K.$ \sigma =\dfrac{\overline{{\rho }_{w}}}{\overline{{\rho }_{a}}} $ ,$ {\rho }_{a}=\dfrac{P-e}{{R}_{a}T} $ is the dry air density; the saturated water vapor pressure$ e={\rho }_{w}{R}_{w}T $ ; the dry air gas constant is$ {R}_{a}=\dfrac{R}{28.965} $ , and the water vapor gas constant is$ {R}_{w}=461.25 $ .3.3 Quality Assessment
In this section, the observed data were subjected to turbulence spectral analysis, a turbulence smoothness test, and a turbulence development adequacy test; the data quality was classified via Mauder’s grading criteria (Mauder and Foken, 2015) (Table 3).
Table 3. Turbulence data quality classification standards.Turbulence stability (%) Turbulence development adequacy (%) overall quality level <30 <30 0 <100 <100 1 >100 >100 2 Note: * Level 0 is high quality data that can be used for basic research analysis; Grade 1 is medium quality data, which can be used for general air-sea flux analysis; Level 2 is low-quality data and should be discarded or interpolated. 3.3.1 Turbulence spectrum analysis
The one-dimensional wind speed vector conformed to the “Kolmogorov –5/3” law, i.e., the slope of the inertial subregion satisfied “–5/3” in the case of local uniform isotropy (Li et al., 2021a). To verify the form of energy transport in the entire system of atmospheric turbulence, the power spectrum and co-spectrum models proposed by Kaimal et al. (1972) based on the “Kolmogorov –5/3” law are commonly used. In other words, the power spectrum in the inertial subregion follows the “–2/3” law and the co-spectrum follows the “–4/3” law.
Turbulence spectral analysis is the power and co-spectral analysis of wind speed and water vapor or CO2 (Kaimal et al., 1972; Su et al., 2004):
$$ spectrapower:\frac{nS\left(n\right)}{{u}_{*}^{2}} , $$ (15) $$ cospectra:\frac{nC{o}_{wc}\left(n\right)}{\overline{{w}^{\text{'}}{c}^{\text{'}}}} , $$ (16) where
$ n $ is the natural frequency;$ S\left(n\right) $ is the spectral density; and$ {Co}_{wc}\left(n\right) $ is the co-spectral density;$ {u}_{*}=\big({\overline{{u}{{'}}{w}{{'}}}}^{2}+ {\overline{{u}{{'}}{v}{{'}}}}^{2}\big)^{1/4} $ is the friction velocity.3.3.2 Turbulence stationarity test
To ensure that the half-hourly turbulence statistics were stable, a turbulence smoothness test was performed using the following expression (Lee et al., 2005):
$$ \Delta st=\left|\frac{\overline{({w}{{'}}{c}{{'}}{)}_{5}}-\overline{({w}{{'}}{c}{{'}}{)}_{30}}}{\overline{({w}{{'}}{c}{{'}}{)}_{30}}}\right|\times 100\text{%} , $$ (17) The subscripts “5” and “30” represent 5 and 30 min samples, respectively, in the same time period.
3.3.3 Turbulence development adequacy
The adequacy of turbulence development can be expressed in terms of the overall turbulence characteristic coefficients (ITC, integral turbulence characteristics). Atmospheric turbulence is considered to be adequately developed if the ITC is < 30% (Kaimal et al., 1972; Lee et al., 2005). The ITC expression is:
$$ \text{IT}{\text{C}}_{\sigma }=\left|\frac{({\sigma }_{w}/{u}_{*}{)}_{\text{model}}-({\sigma }_{w}/{u}_{*}{)}_{\text{measured}}}{({\sigma }_{w}/{u}_{*}{)}_{\text{model}}}\right| , $$ (18) where
$ {\sigma }_{\mathrm{w}} $ is the vertical wind speed variance. The subscripts model and measured denote the modeled and actual measurements, respectively. The empirical relationship between vertical wind speed and friction velocity ratio is given by (Panofsky and Dutton, 1984; Foken et al., 2005):$$ {\left(\frac{{\sigma }_{w}}{{u}_{*}}\right)}_{model}=\left\{\begin{array}{cc}1.3(1-3z/L{)}^{1/3}& z/L < -0.1\\ 1.4& -0.1 < z/L < 0.1\\ 1.5& z/L > 0.1\end{array}\right. , $$ (19) where
$ z $ is the set-up height;$ L $ is the Obukhov length;$ z/L $ is the atmospheric stability factor, which is a dimensionless parameter typically ranging from −5 to 5, with positive (negative) values indicating stable (unstable) values, and values approaching 0 indicate the limit of neutral stratification.The Obukhov length
$ L $ is a parameter that indicates the proportional influence of shear force and bouyancy in the generation and consumption of turbulence kinetic energy:$$ L=-\frac{{{u}_{*}}^{3}\theta }{kg\overline{{w}{\prime}{\theta }{\prime}}} , $$ (20) where
$ k $ is von Kármán’s constant,$ g $ is gravitational acceleration, and$ \theta $ is potential temperature.4. Results and discussion
In this study, data from the offshore platform in August 2020 and the island platform data in July 2021 were used as examples to analyze the air-sea flux observations at different frequencies. Three main aspects were included: data calibration, quality assessment, and differences in air-sea flux observed at different frequencies.
4.1 Data calibration
4.1.1 Time delay
The maximum correlation coefficients of ultrasonic wind speed with water vapor and CO2 concentration were analyzed to determine the delay time using the observation data from the offshore platform, respectively (Fig. 5). The results show the delay times of the ultrasonic anemometer with the high-frequency water vapor pulsometer and the high-frequency CO2 pulsometer are −0.1 s and 1 s, respectively.
4.1.2 Coordinate rotation
Figure 6 shows the changes in the 3-D wind speed vector before and after that DR that transforms the 3-D wind speed vector from the ultrasonic anemometer coordinate system to the natural coordinate system. The pre-correction image (a) shows that the vertical wind speed
$ w $ tends to increase as the horizontal wind speed$ u $ and side wind$ v $ decrease. The vertical distance of the measured 3-D wind speed scattering points from the u-v plane shows that$ w $ was generally positive and the direction was upward before correction. After correction (b),$ w $ was uniformly distributed on both sides of the u-v plane so that$ w=0 $ and included upward and downward vertical wind speeds. In addition, the overall vertical distance after correction was smaller than the vertical distance before correction, and the data scatter was uniformly distributed throughout the plane. The results show that the DR method effectively performed the tilt correction and reduced the interference of different wind speed components.4.1.3 Air-sea flux duration
The Ogive curve, which integrates the co-spectrum of the vertical wind speed
$ w $ and physical quantity x from low to high frequencies, was used to verify the adequacy of the air-sea flux averaging duration in capturing all air-sea fluxes. If the Ogive value converges gradually at low frequencies, it indicates that the air-sea flux computation time scale is appropriate (Oncley et al., 1996; Lee et al., 2005).The Ogive curves of downwind and vertical wind speeds plotted for 16 randomly selected days from our July 2021 offshore platform observations, and randomly selecting 1 hour data each day. Each Ogive Curve corresponds to one hour of data from one of the days. We randomly select as much data as possible to ensure that the chosen data can represent the overall data quality. The results in Fig. 7 show that the Ogive value gradually increases over time. When the observation time reaches 1 min, the Ogive value gradually becomes stable; most of the data become completely stable at 5 min, and the Ogive value gradually approaches 1.0 m2/s2 at 30 min. Oncley et al. (1996) analyzed 10 Hz observation samples and found that the optimal observation length was between 5 min and 30 min, and the corresponding natural frequency was 3.3 × 10–3 Hz ~ 5.6 × 10–4 Hz. Unlike previous studies, the air-sea observation frequencies in this study were carried out at 100 Hz, which allowed for the detection of smaller and more frequent changes in turbulence. To analyze the influence of different observation frequencies on the air-sea flux, we examined the observed data of the air-sea fluxes from offshore platforms and islands, with the shortest duration (5 min) in Oncley et al. (1996).
4.1.4 WPL correction
Data in July 10, 2021 were selected as the island observations, which were more comprehensive than the other days, because it was more complete and allowed to analyze flux changes over the course of the day. As mentioned in Section 2.2, the island observations were only high-frequency observations of water vapor. Therefore, in this section, only the air-sea water vapor fluxes were corrected for WPL, and the changes in air-sea flux before and after the correction were analyzed.
Figure 8 shows that in terms of the magnitude of air-sea flux change, the WPL corrected water vapor flux was smaller than the uncorrected water vapor flux, which is consistent with the results of Jentzsch et al. (2021). The difference was that Fig. 8 has two corrected peaks at 09:00 and 19:25 due to the complex island observing environment. In terms of air-sea flux differences, the WPL correction has a significant effect on the calculated values of water vapor fluxes, with a maximum amplitude effect on air-sea fluxes of about 1.5 mmol/(m2·s). The air-sea flux differences before and after the correction varied with time and were significantly different. Between 0:00 and 9:00, the air-sea flux difference showed a clear upward trend; from about 9:00 to 18:10, the air-sea flux difference showed a downward trend accompanied by an increase in amplitude of about 1 h, which maintained a stable air-sea flux difference during the night. The results of the WPL corrections show that temperature and water vapor led to a change in the density of the observed amount, resulting in notable changes in air-sea flux change. The faster the temperature and water vapor changed, the larger the magnitude of the correction.
4.2 Quality assessment
4.2.1 Turbulence spectrum analysis
The wind speed power spectrum and its co-spectrum with CO2 and water vapor are analyzed from selected island observations. Fig. 9 shows that the inertial subregion was in the frequency range of approximately 1 Hz to 10 Hz. The peak value of the power spectrum appears at a frequency lower than 1 Hz, representing a region of high energy content known as the energy-containing vortex region, and the spectrum value in the range from 10–3 to 100 increases with the increase of frequency. When the frequency is about 1 Hz to 10 Hz, the power spectrum shows a downward trend, and the turbulent kinetic energy produces a turbulent cascade with isotropic characteristics. Parallel to the slope line, this part follows the −2/3 law, representing the inertial subregion. When the frequency is higher than 10 Hz, the spectral value change deviates from this slope line, which indicates the onset of the dissipation region. Here, turbulent kinetic energy is transferred to a smaller-scale vortex and dissipates into internal energy due to molecular viscosity.
In stable conditions (–0.1 < z/L≤ 1) at low frequencies, data from offshore platforms shows an upward trend as frequency decreases, while the island data shows a decreasing trend with the decrease of frequency. This opposite trend between the two instruments indicates that the 100 Hz instruments are more capable of capturing detailed changes in the low-frequency region.
Figure 10 shows the co-spectrum of vertical wind speed
$ w $ with CO2 and water vapor. The solid gray line indicates co-spectra of w with H2O (Fig. 10a) and CO2 (Fig. 10b) respectively. And “●” indicates averaged co-spectra data. The gray shading in the Fig. shows the relationship between the raw data and the frequency, and the black dots are quality-controlled averages. In calculating the averaged co-spectra data logarithms with e as the base, while the overall representation of the image is a coordinate system with 10 as the base, which better presents the trend of the data. As shown in Fig. 10, the distribution of the mean values is relatively uniform, and the entire result shows a trend that is generally consistent with the –4/3 slope, especially between frequencies greater than 100 Hz and less than 102 Hz.Figure 10. Co-spectral analysis. (a), (b) are co-spectra of$ w $ with H2O and CO2, respectively. The solid gray line indicates co-spectra of$ w $ with H2O (Fig. 10a) and CO2 (Fig. 10b) respectively. And “●” indicates averaged co-spectra data, the dashed line indicates the “–4/3” slope; the solid black line indicates the Kaimal et al. (1972) is suitable for atmospheric stabilization.The measured mean data were most consistent with the Kaimal et al. (1972) atmospheric instability (–1.0 < z/L ≤ –0.1) in the low-frequency region, and the trend of the scatter distribution in the high-frequency region was more consistent with the –4/3 slope. At frequencies less than 0.01 Hz, the co-spectral values were higher than those of Kaimal’s results due to factors such as insufficient sample data and noise. At frequencies higher than 10 Hz, small co-spectral densities may lead to larger measurement errors (Su et al., 2004). Combining the power spectrum and co-spectrum results, the high-frequency observations fulfil the power spectrum slope of –2/3 and the co-spectrum slope of –4/3 in the inertial sub-region.
4.2.2 Overall quality assessment
Due to the short time series of the offshore platform observation data, which is not conducive to turbulence quality analysis, the island observation data were used to evaluate the overall turbulence quality of the air-sea fluxes and assess the overall quality of the water vapor flux data with three grades of 0, 1, and 2 (see Table 3).
Figure 11 shows the overall turbulence data quality after the turbulence smoothness test and the turbulence development adequacy test. The results show that high-quality data account for about 46.5% of the overall air-sea flux data, and 25.0% of the moderate-quality data. About 28.5% of the points to be discarded were distributed around 12:00 on July 10 and 11. Overall about 71.5% of the island water vapor flux data passed the turbulence smoothness test and the turbulence development adequacy test. In Fig. 11 around 14:00 on July 11, there is a data point with a value of 5 mmol/(m2·s) and QC = 1, which means that this is the general quality data, and around 20:00 in the evening of July 11, the water vapor flux has a value of –3 mmol/(m2·s) and QC = 2, which means that it is the low-quality data. It can be seen that the QC = 1 data interval used for the general quality analysis is quite scattered, with a large difference between the maximum and minimum values.
4.3 High/low frequency comparison
Figure 12 shows the air-sea flux observations from the offshore platform. The 100 Hz and 20 Hz air-sea flux trends were generally the same but with larger variations. Water vapor fluxes showed opposite trends for the high and low-frequency observations from 14:55 to 15:05 (Fig. 12a). This may be related to the fact that more turbulent changes are monitored by high-frequency observations, and it is normal for air-sea flux trends to be reversed on smaller timescale scales. At 16:45, the CO2 flux had a negative air-sea flux in high-frequency measurements and a positive flux in low-frequency measurements (Fig. 12b). These results indicate that the measurement frequency had an important effect on the air-sea flux, affecting both intensity and direction.
Figure 13a shows the difference between the high and low-frequency water vapor fluxes. These air-sea fluxes were small and less than 0.01 mmol/(m2·s) on July 9, while the difference between the fluxes on July 10 and 11 increased from about 0.005 to 0.03 mmol/(m2·s). According to the experimental record, there was rain on the island on July 10 and 11, which increased the water vapor density in the air and subsequently the air-sea fluxes. Figs. 13b, c, and d show the local magnification of the water vapor flux variations on July 9, 10, and 11, respectively. During this time, the water vapor fluxes varied between –0.2 and 1.00 mmol/(m2·s), with total air-sea fluxes being positive. From 15:21 to 15:43 on July 11, the air-sea flux peaks were lower in the high-frequency observations than in the low-frequency observations. An opposite trend was observed at 15:00 observation at the offshore platform (Fig. 12a). These two fluxes are not directly correlated and have different response rates for different concentrations in air, the source-sink variations in H2O and CO2 fluxes are not in one-to-one correspondence. During the field measurements, the weather and instrument shaking conditions were the same, and the performance was stable except for a sudden drop in the CO2 values at 15:00. This inconsistency in the sudden change may be due to the fact that the two fluxes were measured at some distance from each other in the field, which had a certain impact on the results. In addition to this, it can also be shown that this difference was caused by high-frequency observations that were able to capture additional information about turbulence changes, both upward and downward, on a different timescale. Taken together, the frequency of the observations had a smaller effect on the water vapor flux than on the CO2 flux, probably because the water vapor concentration in the atmosphere was much higher than the CO2 concentration.
Figure 13. Comparison of observed air-sea fluxes at different frequencies over the island. (a) Difference between 100 Hz and 20 Hz air-sea fluxes; (b), (c), and (d) are partial zoomed-in plots showing island air-sea flux observations on July 9, 10, and 11, 2021, respectively. The “//” denotes the axes of the truncated, partially invalid data, “▼” denotes 100 Hz, and “●” denotes 20 Hz.Figure 14 shows the evolution over time of water vapor and CO2 concentrations. The high-frequency observations had more pulsed values and more pronounced amplitudes of change than the low-frequency observations. Observations from the offshore platform were smooth, with high water vapor concentrations and narrow variations. Long-term observations on the islands showed some cyclical variations in water vapor concentration, with a sudden drop in water vapor concentration on July 11. This systematic and abrupt change can be attributed to factors such as changes in meteorological conditions and sensor drift (Moncrieff et al., 2004). The change in the trend of the data from the offshore platforms was not as obvious. There is a downward trend in the data from the platforms on the islands. In terms of the overall performance of the data, the high-frequency observations were able to monitor more of the small changes in the turbulence than the low-frequency data.
Figure 14. Water vapor and CO2 concentrations over time; (a) and (b) are water vapor versus time for offshore platform and island data, respectively. The inner image of (a) is its local zoom; (c) is the CO2 variation with time. The red solid line indicates 100 Hz observations and the black solid line indicates 20 Hz observations.Figure 15 comprehensively shows the variation of air-sea fluxes between the island and offshore platforms with wind speed. Despite higher overall wind speeds at the offshore compared to that of the offshore platform, their air-sea fluxes show opposite behaviors. One difference is that the air-sea flux varies in size; second, the trends in air-sea flux change differ. These air-sea fluxes are determined by two factors: wind speed and gas concentration. In the nearshore seas, the proximity to land promotes frequent gas exchange, resulting in a higher variability in air-sea flux values. The air-sea flux on the offshore platform decreases with increasing wind speed, while on the island, air-sea flux increases with the increase of wind speed. These results show that with higher wind speeds, the island environment has more vertical turbulence than the offshore platform, leading to increased atmospheric instability increases. As wind speed increases, the error in air-sea flux measurements also increases. There is no significant difference observed in measurement errors between high-frequency and low-frequency measurements. However, as wind speed increases, the difference in air-sea flux between the high and low-frequency measurements on the two platforms becomes more pronounced.
In Fig. 16, it is evident that water vapor fluxes from offshore platforms exhibit an inverse trend to CO2 fluxes, and opposite to the variation trend of water vapor flux on the islands. The water vapor flux of the offshore platform decreases with the increase in wind speed, and the water vapor flux value ranges from −0.1 mmol/(m2·s) to 0.2 mmol/(m2·s), while the wind speed ranges from 8 m/s to 11 m/s, indicating low and intermediate wind speeds. The ambient water vapor flux ranges from −0.2 mmol/(m2·s) to 1 mmol/(m2·s), and the wind speed ranges from 0 m/s to 8 m/s. The overall water vapor flux direction of the island environment trends upward, while the water vapor flux of the offshore platform is balanced and the water vapor exchange is weak. For CO2 flux, the overall air-sea flux direction trends upward, and the ocean is the source of CO2 release. In obtaining this result, we did not take into account the problem of the distance from the installation position of the instrument, and we hope to further improve the accuracy of our results in subsequent experiments. In terms of basic variation trends and errors, the results in Fig. 16 are consistent with those in Fig.15.
The above results show that the trends of variation in air-sea flux with wind speeds are notably different due to differences in the underlying surface environment and atmospheric stability. With the increase of wind speed, the air-sea flux measurement error increases, so the uncertainty of observation frequency to air-sea flux increases. However, the overall difference between 100 Hz and 20 Hz errors is not large, and the observed air-sea flux values are different.
There are few reports on gas analyzers at 100 Hz, and there is no fixed relationship between air-sea CO2 and water vapor flux and wind speed. This is because wind speed mainly changes the transfer velocity without altering the magnitude of the partial pressures of CO2 or water vapor. The carbon source or sink primarily depends on the difference in CO2 partial pressure between the atmosphere and seawater. If the atmospheric CO2 partial pressure is greater, the ocean acts as a carbon sink, and if the ocean's CO2 partial pressure is greater, the ocean acts as a carbon source. The difference in CO2 partial pressure varies across different regions, hence the relationship between flux and wind speed will differ. This paper mainly aims to illustrate the differences in air-sea CO2 and water vapor flux observed at 20 Hz and 100 Hz, as well as the fact that high-frequency observations are superior to low-frequency observations under high wind speed conditions, through Fig. 16. The work of Li et al. (2021b) can support our conclusion.
Changes in turbulence occur very quickly, and thus concentrations and densities change accordingly. It is therefore necessary to use instruments with high accuracy and fast measurement data transfer rates, especially in windy environments. (1) Due to the limitation of low frequency of observation instruments, it often happens that the inertial domain band of the measured spectrum is too narrow to fit the Kolmogorov-5/3 power law well. The turbulence energy spectrum obtained from high-frequency observation covers not only the inertial subregion, but also part of the viscous subregion, which is conducive to calculating the turbulence parameters more accurately, and to identifying and analyzing turbulence characteristics. (2) When using Ogive Curves to calculate the averaging time, low-frequency observations may mask or smooth out some transient features of turbulence, while high-frequency observations can reduce this effect and make the observations closer to the real situation. Also, due to the intermittent nature of turbulence, turbulent and non-turbulent layers that are clearly bounded in low-frequency observations may underestimate certain fluxes. High-frequency observations can reduce this underestimation by capturing more turbulent activity, estimating turbulent fluxes more accurately, and tracking transient changes in turbulence. In addition to this, high-frequency observations provide data with higher temporal resolution, which page means that rapid changes in turbulence over time can be observed in more detail.
5. Conclusion
In this paper, we used water vapor and CO2 flux observations from offshore platforms and island platforms to carry out comprehensive data calibration and data quality, focusing on the effects of 100 and 20 Hz observing frequencies on air-sea flux observations. Different durations between different frequencies were found to have a great influence on the air-sea flux calculations. We chose a duration of 5 min for these calculations. Based on the results of the turbulence spectrum analysis, turbulence smoothness, and turbulence development adequacy tests, the observed data met the requirements for accurate air-sea flux calculations. The temperature and water vapor density corrections reduced the air-sea flux magnitude to some extent, and further reduced the air-sea flux differences caused by different observation frequencies. At low wind speeds, there were more high-frequency observations with different air-sea flux magnitudes and directions than low-frequency observations. The frequency of the wind speed observations was not the main factor contributing to the air-sea flux differences; rather, it was the magnitude of wind speed that contributed to the differences between high and low-frequency observations. At high wind speeds, the difference between high and low-frequency water vapor fluxes was small, and there was an overall large difference in CO2 fluxes. The results regarding the effects of environmental factors such as wind speed, temperature, and water vapor on the air-sea fluxes under high-frequency and low-frequency observations showed that high-frequency observations could provide more detailed information on turbulence variations than low-frequency data. Furthermore, changes in the air-sea fluxes of gases with lower concentrations in the atmosphere could be observed on short time scales.
-
Figure 10. RDA triplots depicting the interrelationships between MZ biomass and abundance of dominant taxa, and environmental variables in the nearshore waters of Mangalore (a) and the NGES (b). Sampling stations are indicated by blue circles; environmental parameters are represented by red arrows; MZ parameters (Bio-Biomass, Cpd-Copepod, Cdc-Cladocera, Dcp-Decapoda larvae, Luc-Lucifer, Ctg-Chaetognatha, Bvl-Bivalve larvae, Npl-Nauplius larvae, Cpl-Copepodite) are represented by green arrows.
Figure 11. RDA triplots depicting the interrelationships between major copepod species and environmental variables in the nearshore waters of Mangalore (a) and the NGES (b). Sampling stations are indicated by blue circles; environmental parameters are represented by red arrows; Copepods (BS-Bestiolina similis, PSA-Pseudodiaptomus aurivillii, CC-Onychocorycaeus catus, TT-Temora turbinata, EA-Euterpina acutifrons, UV-Undinula vulgaris AD-Acartia danae, CF-Centropages furcatus, PS-Pseudodiaptomus serricaudatus) are represented by green arrows.
Table 1. Mean abundance of copepod species in the nearshore waters of Mangalore and NGES during winter monsoon
Copepod species Nearshore Estuary Order calanoida Bestiolina similis **** * Pseudodiaptomus aurivillii ** * Acartia erythraea ** - Acartia danae ** * Centropages furcatus ** * Temora turbinata ** - Undinula vulgaris * * Acrocalanus gracilis * * Labidocera pectinata * - Calanopia minor * - Labidocera minuta * - Pseudodiaptomus serricaudatus ** * Acartia spinicauda * * Centropages orsinii * - Centropages calaninus * - Acartia southwelli - * Subeucalanus crassus * - Labidocera acuta * - Paracalanus aculeatus - * Calocalanus pavo - * Order Poecilostomatoida Onychocorycaeus catus *** * Oncaea venusta * - Sapphirina sp. * * Order Harpacticoida Euterpina acutifrons * * Miracia efferata * - Order Cyclopoida Oithona similis * * Note: * <100, ** 100−500, *** 500− 1000 , and ****1000 −2000 .Table 2. Mean abundance of various mesozooplankton taxa in the nearshore waters of Mangalore and NGES during winter monsoon
MZ taxa Nearshore NGES Copepods 4491 ±2605 76.7±130.4 Crustacean larvae Copepodites 204±297 0 Zoea 4±5.3 0.8±1 Nauplii 32±66 119.3±216.5 Decapod larvae 506±313 10±12.7 Megalopa larvae 1.7±7.2 0 Cladocerans 1059 ±8260.8±1.4 Ostracods 0 0.2±0.3 Chaetognaths 59.1±32 0.1±0.2 Ichthyoplankton Fish larvae 0.7±1.5 0.0±0.1 Fish eggs 4.8±7.5 0.7±0.9 Molluscan larvae Bivalve larvae 161.3±348 16.5±24.7 Decapods Lucifer sp. 365±325 0.8±1.5 Pelagic tunicates Oikopleura sp. 11.8±18 0.1±0.3 Ctenophors 0 0.1±0.1 Hydrozoa 0.4±0.7 0.0±0.1 Polychaetes 2.5±6 0.2±0.6 Table 3. Major copepod Characterizing species in the nearshore waters of Mangalore and NGES during winter monsoon
Species Average Abundance Average Similarity Similarity/SD Contribution/% Cumulative/% Nearshore Bestiolina similis 6.11 13.45 3.56 20.31 20.31 Onychocorycaeus catus 4.68 10.39 4.35 15.69 36 Pseudodiaptomus aurivillii 4.13 9.34 4.73 14.11 50.11 Acartia danae 3.43 7.33 2.61 11.08 61.19 Centropages furcatus 3.28 6.9 2.63 10.42 71.61 Temora turbinata 3.1 5.25 1.18 7.93 79.54 NGES Bestiolina similis 1.72 11.67 1.04 40.5 40.5 Table 4. Description of feeding guilds of major MZ taxa and copepods observed in the near shore waters of Mangalore and NGES
Mesozooplankton Feeding guilds Reference Copepod species Order Calanoida Bestiolina similis* herbivore Deb Bhattacharya et al. (2015) Pseudodiaptomus aurivillii* carnivore Jitchum and Wongrat (2009) Acartia erythraea# omnivore Jitchum and Wongrat (2009) Centropages furcatus* omnivore Marshall and Orr (1966) Temora turbinata# herbivore Ikeda (1977); Turner (1984); Deb Bhattacharya et al. (2015) Acrocalanus gracilis* herbivore Nandy et al. (2018) Labidocera pectinata# carnivore Jitchum and Wongrat (2009) Labidocera minuta# herbivore Nandy et al. (2018) Pseudodiaptomus serricaudatus* herbivore Deb Bhattacharya et al. (2015) Acartia spinicauda* omnivore Deb Bhattacharya et al. (2015); Nandy et al. (2018) Centropages orsinii# omnivore Turner (1984); Calbet et al. (2007); Jitchum and Wongrat (2009) Acartia southwelli+ omnivore Nandy et al. (2018) Labidocera acuta# herbivore Nandy et al. (2018) Paracalanus aculeatus+ herbivore Nandy et al. (2018); Jitchum and Wongrat (2009) Subeucalanus crassus# herbivore Wickstead (1962); Paffenhöfer et al. (1982) Order Poecilostomatoida Onychocorycaeus catus* carnivore Jitchum and Wongrat (2009) Oncaea venusta# omnivore Turner (1986) Sapphirina sp.* carnivore Heron (1973); Jitchum and Wongrat (2009) Order Harpacticoida Euterpina acutifrons* detritivore Deb Bhattacharya et al. (2015); Nandy et al. (2018) Order Cyclopoida Oithona similis* carnivore Deb Bhattacharya et al. (2015) Note: * Both, # nearshore, and + estuary. Table 5. Results of one-way ANOVA of major physico-chemical and biological parameters between the nearshore waters and NGES
Parameters F P-value Temperature/℃ 0.00 0.9641 Salinity* 64.02 0.0000 Turbidity (NTU) 1.75 0.1940 Dissolved oxygen/mg·L−1 0.81 0.3748 Nitrite/μmol·L−1 * 21.99 0.0000 Nitrate/μmol·L−1 * 6.90 0.0126 Ammonium/μmol·L−1 * 22.07 0.0000 Phosphate/μmol·L−1 * 7.42 0.0099 Silicate/μmol·L−1 * 59.19 0.0000 Chl a/mg·m−3 0.12 0.7360 Mesozooplankton biomass/mL·m−3 * 82.76 0.0000 Mesozooplankton abundance/ind.·m−3 * 49.23 0.0000 Copepod abundance/ind.·m−3 * 36.65 0.0000 Bestiolina similis abundance/ind.·m−3 * 14.51 0.0006 Note: * The significant variation at 5% level (P<0.05). -
Achuthankutty C T, Ramaiah N, Padmavati G. 1998. Zooplankton variability and copepod assemblage in the coastal and estuarine waters of Goa along the central-west coast of India. IOC Workshop Report No. 142 Anandavelu I, Robin R S, Purvaja R, et al. 2020. Spatial heterogeneity of mesozooplankton along the tropical coastal waters. Continental Shelf Research, 206: 104193, doi: 10.1016/j.csr.2020.104193 Andrade F, Aravinda H B, Puttaiah E T. 2011. Studies on Mangalore coastal water pollution and its sources. Indian Journal of Science and Technology, 4(5): 553–557, doi: 10.17485/ijst/2011/v4i5.26 Arunpandi N, Jyothibabu R, Jagadeesan L, et al. 2020. Impact of a large hydraulic barrage on the trace metals concentration in mesozooplankton in the Kochi backwaters, along the Southwest coast of India. Marine Pollution Bulletin, 160: 111568, doi: 10.1016/j.marpolbul.2020.111568 Baliarsingh S K, Lotliker A A, Srichandan S, et al. 2020. A review of jellyfish aggregations, focusing on India’s coastal waters. Ecological Processes, 9(1): 58, doi: 10.1186/s13717-020-00268-z Baretta J W, Malschaert J F P. 1988. Distribution and abundance of the zooplankton of the Ems estuary (North Sea). Netherlands Journal of Sea Research, 22(1): 69–81, doi: 10.1016/0077-7579(88)90053-1 Bhat B V, Gupta T R C. 1983. Zooplankton distribution in Nethravati-Gurupur Estuary, Mangalore. Indian Journal of Marine Sciences, 121: 36–42 Calbet A, Carlotti F, Gaudy R. 2007. The feeding ecology of the copepod Centropages typicus (Kröyer). Progress in Oceanography, 72(2-3): 137–150, doi: 10.1016/j.pocean.2007.01.003 Calbet A, Landry M R. 1999. Mesozooplankton influences on the microbial food web: direct and indirect trophic interactions in the oligotrophic open ocean. Limnology and Oceanography, 44(6): 1370–1380, doi: 10.4319/lo.1999.44.6.1370 Cardoso P G, Raffaelli D, Lillebø A I, et al. 2008. The impact of extreme flooding events and anthropogenic stressors on the macrobenthic communities’ dynamics. Estuarine, Coastal and Shelf Science, 76(3): 553–565, doi: 10.1016/j.ecss.2007.07.026 Chen Mianrun, Liu Hongbin, Chen Bingzhang. 2017. Seasonal variability of mesozooplankton feeding rates on phytoplankton in subtropical coastal and estuarine waters. Frontiers in Marine Science, 4: 186, doi: 10.3389/fmars.2017.00186 Chew L L, Chong V C. 2011. Copepod community structure and abundance in a tropical mangrove estuary, with comparisons to coastal waters. Hydrobiologia, 666(1): 127–143, doi: 10.1007/s10750-010-0092-3 Clarke K R, Gorley R N. 2015. Getting started with PRIMER v7. PRIMER-E: plymouth. Plymouth Marine Laboratory Clarke K R, Warwick R M. 2001. Changes in Marine Communities: An Approach to Statistical Analysis and Interpretation. 2nd ed. PRIMER-E: Plymouth Cloern J E, Foster S Q, Kleckner A E. 2014. Phytoplankton primary production in the world's estuarine-coastal ecosystems. Biogeosciences, 11(9): 2477–2501, doi: 10.5194/bg-11-2477-2014 Conway D V P, White R G, Hugues-Dit-Ciles J, et al. 2003. Guide to the coastal and surface zooplankton of the south-western Indian Ocean. Plymouth: Marine Biological Association of the United Kingdom, 1–354 Costanza R, d'Arge R, de Groot R, et al. 1997. The value of the world's ecosystem services and natural capital. Nature, 387(6630): 253–260, doi: 10.1038/387253a0 Dalal S G, Goswami S C. 2001. Temporal and ephemeral variations in copepod community in the estuaries of Mandovi and Zuari—west coast of India. Journal of Plankton Research, 23(1): 19–26, doi: 10.1093/plankt/23.1.19 David V, Sautour B, Chardy P, et al. 2005. Long-term changes of the zooplankton variability in a turbid environment: the Gironde estuary (France). Estuarine, Coastal and Shelf Science, 64(2–3): 171–184, doi: 10.1016/j.ecss.2005.01.014 Deb Bhattacharya B, Bhattacharya A K, Rakshit D, et al. 2014. Impact of the tropical cyclonic storm ‘Aila’ on the water quality characteristics and mesozooplankton community structure of Sundarban mangrove wetland, India. Indian Journal of Geo-Marine Sciences, 43(2): 216–223 Deb Bhattacharya B, Hwang J S, Sarkar S K, et al. 2015. Community structure of mesozooplankton in coastal waters of Sundarban mangrove wetland, India: a multivariate approach. Journal of Marine Systems, 141: 112–121, doi: 10.1016/j.jmarsys.2014.08.018 Devaraj M, Vivekanandan E. 1999. Marine capture fisheries of India: challenges and opportunities. Current Science, 76(3): 314–332 Durga Rao G, Kanuri V V, Kumaraswami M, et al. 2017. Dissolved nutrient dynamics along the southwest coastal waters of India during northeast monsoon: a case study. Chemistry and Ecology, 33(3): 229–246, doi: 10.1080/02757540.2017.1287903 Gayathri S, Krishnan K A, Krishnakumar A, et al. 2021. Monitoring of heavy metal contamination in Netravati river basin: overview of pollution indices and risk assessment. Sustainable Water Resources Management, 7(2): 20, doi: 10.1007/s40899-021-00502-2 Gowda G, Gupta T R C, Rajesh K M, et al. 2002. Distribution of phytoplankton pigments in the Nethravathi estuary. Indian Journal of Fisheries, 49(3): 267–273 Grasshoff K, Erhardt M, Kremling K. 1983. Methods of Seawater Analysis. 2nd ed. Weiheim: Verlag Chemie, 61–72 Harfmann J, Kurobe T, Bergamaschi B, et al. 2019. Plant detritus is selectively consumed by estuarine copepods and can augment their survival. Scientific Reports, 9(1): 9076, doi: 10.1038/s41598-019-45503-6 Harris R, Wiebe P, Lenz J, et al. 2000. ICES Zooplankton Methodology Manual. San Diego: Academic Heron A C. 1973. A specialized predator-prey relationship between the copepod Sapphirina angusta and the pelagic tunicate Thalia democratica. Journal of the Marine Biological Association of the United Kingdom, 53(2): 429–435, doi: 10.1017/S0025315400022372 Ikeda T. 1977. Feeding rates of planktonic copepods from a tropical sea. Journal of Experimental Marine Biology and Ecology, 29(3): 263–277, doi: 10.1016/0022-0981(77)90070-3 Jagadeesan L, Jyothibabu R, Arunpandi N, et al. 2017. Copepod grazing and their impact on phytoplankton standing stock and production in a tropical coastal water during the different seasons. Environmental Monitoring and Assessment, 189(3): 105, doi: 10.1007/s10661-017-5804-y Jitchum P, Wongrat L. 2009. Community structure and abundance of epipelagic copepods in a shallow protected bay, Gulf of Thailand. Journal of Fisheries and Environment, 33(1): 28–40 Jyothibabu R, Madhu N V. 2007. Zooplankton in the Mandovi and Zuari estuary. In: Mandovi and Zuari estuaries eds. (SR. Shyte, M. Dileep kumar and D. Shankar). 83–90 Karati K K, Ashadevi C R, Harikrishnachari N V, et al. 2021. Hydrodynamic variability and nutrient status structuring the mesozooplankton community of the estuaries along the west coast of India. Environmental Science and Pollution Research, 28(31): 42477–42495, doi: 10.1007/s11356-021-13634-x Karthikeyan P, Marigoudar S R, Mohan D, et al. 2020. Ecological risk from heavy metals in Ennore estuary, South East coast of India. Environmental Chemistry and Ecotoxicology, 2: 182–193, doi: 10.1016/j.enceco.2020.09.004 Kasturirangan L R. 1963. Key for the identification of the more common planktonic copepoda of Indian coastal waters. In: Key for the Identification of the More Common Planktonic Copepoda of Indian Coastal Waters. New Delhi: The Council of Scientific and Industrial Research, 1–92 Kennish M J. 1990. Ecology of Estuaries Volume 2: Biological Aspects. Boca Raton: CRC Press, doi: 10.1201/9781351071604 Ketchum B H. 1983. Estuaries and Enclosed Seas. Amsterdam: Elsevier Krishnakumar P K, Bhat G S. 2008. Seasonal and interannual variations of oceanographic conditions off Mangalore coast (Karnataka, India) in the Malabar upwelling system during 1995–2004 and their influences on the pelagic fishery. Fisheries Oceanography, 17(1): 45–60, doi: 10.1111/j.1365-2419.2007.00455.x Kumar M R, Krishnan K A. 2021. Grazing behaviour of tropical calanoid copepods and its effect on phytoplankton community structure. Environmental Monitoring and Assessment, 193(8): 495, doi: 10.1007/s10661-021-09306-5 Kumar M R, Krishnan K A, Das R, et al. 2020. Seasonal phytoplankton succession in Netravathi–Gurupura estuary, Karnataka, India: study on a three tier hydrographic platform. Estuarine, Coastal and Shelf Science, 242: 106830, doi: 10.1016/j.ecss.2020.106830 Lancelot C, Muylaert K. 2011. Trends in estuarine phytoplankton ecology. In: Wolanski E, ed. Treatise on Estuarine and Coastal Science. Amsterdam: Academic Press, 5–15 Lee C Y, Liu D C, Su W C. 2009. Seasonal and spatial variations in the planktonic copepod community of Ilan Bay and adjacent Kuroshio waters off northeastern Taiwan. Zoological Studies, 48(2): 151–161 Madhu N V, Jyothibabu R, Balachandran K K, et al. 2007. Monsoonal impact on planktonic standing stock and abundance in a tropical estuary (Cochin backwaters–India). Estuarine, Coastal and Shelf Science, 73(1–2): 54–64, doi: 10.1016/j.ecss.2006.12.009 Madhu N V, Martin G D, Haridevi C K, et al. 2017. Differential environmental responses of tropical phytoplankton community in the southwest coast of India. Regional Studies in Marine Science, 16: 21–35, doi: 10.1016/j.rsma.2017.07.004 Madhupratap M. 1987. Status and strategy of zooplankton of tropical Indian estuaries: a review. Bulletin of Plankton Society of Japan, 34(1): 65–81 Manjunatha B R, Shankar R. 2000. Behaviour and distribution patterns of particulate metals in estuarine and coastal surface waters near Mangalore, southwest coast of India. Journal of the Geological Society of India, 55(2): 157–166 Marazzo A, Valentin J L. 2003. Population dynamics of Penilia avirostris (Dana, 1852) (Cladocera) in a tropical bay. Crustaceana, 76(7): 803–817, doi: 10.1163/15685400360730598 Mariottini G L, Pane L. 2010. Mediterranean jellyfish venoms: a review on scyphomedusae. Marine Drugs, 8(4): 1122–1152, doi: 10.3390/md8041122 Marshall S M, Orr A P. 1966. Respiration and feeding in some small copepods. Journal of the Marine Biological Association of the United Kingdom, 46(3): 513–530, doi: 10.1017/S0025315400033312 Maas O. 1907. Die Scyphomedusen. Ergebn Fortsch Zool II: 13–238. https://doi.org/10.5962/bhl.title.11300 Musialik-Koszarowska M, Dzierzbicka-Głowacka L, Weydmann A. 2019. Influence of environmental factors on the population dynamics of key zooplankton species in the Gulf of Gdańsk (southern Baltic Sea). Oceanologia, 61(1): 17–25, doi: 10.1016/j.oceano.2018.06.001 Nandy T, Mandal S, Chatterjee M. 2018. Intra-monsoonal variation of zooplankton population in the Sundarbans Estuarine System, India. Environmental Monitoring and Assessment, 190(10): 603, doi: 10.1007/s10661-018-6969-8 Nayar S, Gupta T R C, Prabhu H V. 2001. Bloom of Noctiluca scintillans MaCartney in the arabian sea off mangalore southwest India. Asian Fisheries Science, 14(1): 77–82, doi: 10.33997/j.afs.2001.14.1.008 Odum E P. 1971. Fundamentals of Ecology. 3rd ed. Philadelphia: Saunders Øresland V. 1987. Feeding of the chaetognaths Sagitta elegans and S. setosa at different seasons in Gullmarsfjorden, Sweden. Marine Ecology Progress Series, 39(1): 69–79 Padmavati G, Goswami S C. 1996. Zooplankton ecology in the Mandovi-Zuari estuarine system of Goa, west coast of India. Indian Journal of Marine Sciences, 25(3): 268–273 Paffenhöfer G A, Lewis K D. 1990. Perceptive performance and feeding behavior of calanoid copepods. Journal of Plankton Research, 12(5): 933–946, doi: 10.1093/plankt/12.5.933 Paffenhöfer G A, Strickler J R, Alcaraz M. 1982. Suspension-feeding by herbivorous calanoid copepods: a cinematographic study. Marine Biology, 67(2): 193–199, doi: 10.1007/BF00401285 Panikkar N K, Aiyar R G. 1939. Observations on breeding in brackish-water animals of Madras. Proceedings/Indian Academy of Sciences, 9(6): 343–364, doi: 10.1007/BF03048507 Paul S, Karan S, Deb Bhattacharya B. 2020. Daily variability of copepods after successive tropical cyclones in the Ganges River estuary of India. Estuarine, Coastal and Shelf Science, 246: 107048, doi: 10.1016/j.ecss.2020.107048 Perissinotto R, Stretch D D, Whitfield A K, et al. 2010. Ecosystem functioning of temporarily open/closed estuaries in southafrica. In: Crane J R, Solomon A E, eds. Estuaries: Types, Movement Patterns and Climatical Impacts. Hauppauge: Science Publishers Price H J. 1988. Feeding mechanisms in marine and freshwater zooplankton. Bulletin of Marine Science, 43(3): 327–343 Primo A L, Azeiteiro U M, Marques S C, et al. 2009. Changes in zooplankton diversity and distribution pattern under varying precipitation regimes in a southern temperate estuary. Estuarine, Coastal and Shelf Science, 82(2): 341–347. doi: 10.1016/j.ecss.2009.01.019 Purcell J E, Uye S I, Lo W T. 2007. Anthropogenic causes of jellyfish blooms and their direct consequences for humans: a review. Marine Ecology Progress Series, 350: 153–174, doi: 10.3354/meps07093 Rajasilta M, Mankki J, Ranta-Aho K, et al. 1999. Littoral fish communities in the Archipelago Sea, SW Finland: a preliminary study of changes over 20 years. Hydrobiologia, 393: 253–259, doi: 10.1023/A:1003523308309 Rajesh M, Rajesh KM, Reddy H RV. 2014. Assessment of Microbial population in relation with hydrographical characteristics in Netravati and Gurupur Estuaries off Mangalore, South-West coast of India. The Ecoscan, 8(3-4): 253–256 Raymont J E G. 1983. Plankton and Productivity in the Oceans. 2nd ed. Oxford: Pergamon Press Reddy M P M. 1979. Sediment movement and siltation in the navigational channel of the Old Mangalore port. Proceedings of the Indian National Science Academy, 88: 121–130 Resmi S, Reddy R V, Venkatesha Moorthy K S, et al. 2011. Zooplankton dynamics in the coastal waters of adubidri, Karnataka. Indian Journal of Geo-Marine Sciences, 40(1): 134–141 Rivonker C U, Verlencar X N, Reddy M P M. 1990. Physico-chemical characteristics of fishing grounds off Mangalore, west coast of India. Indian Journal of Marine Sciences, 19: 201–205 Rose T H, Tweedley J R, Warwick R M, et al. 2019. Zooplankton dynamics in a highly eutrophic microtidal estuary. Marine Pollution Bulletin, 142: 433–451, doi: 10.1016/j.marpolbul.2019.03.047 Santhakumari V, Nair VR. 1999. Distribution of hydromedusae from the exclusive economic zone of the west and east coasts of India. Indian Journal of Marine Sciences, 28: 150–157 Schelske C L, Odum E P. 1962. Mechanisms maintaining high productivity in Georgia estuaries. Proceedings of the Gulf Caribbean Fisheries Institute, 14: 75–80 Schneider G, Behrends G. 1998. Top-down control in a neritic plankton system by Aurelia aurita medusae—a summary. Ophelia, 48(2): 71–82, doi: 10.1080/00785236.1998.10428677 Schultz M, Kiørboe T. 2009. Active prey selection in two pelagic copepods feeding on potentially toxic and non-toxic dinoflagellates. Journal of Plankton Research, 31(5): 553–561, doi: 10.1093/plankt/fbp010 Sewell R B S. 1999. The Copepoda of Indian Seas. Vol 10. Daya Books, Biotech Books, Delhi Shankar R, Manjunatha B R. 1994. Elemental composition and particulate metal fluxes from Netravati and Gurpur rivers to the coastal Arabian Sea. Journal of the Geological Society of India, 43(3): 255–265, doi: 10.17491/jgsi/1994/430304 Shetye S R, Gouveia A D, Shenoi S S C, et al. 1991. The coastal current off western India during the northeast monsoon. Deep-Sea Research Part A. Oceanographic Research Papers, 38(12): 1517–1529, doi: 10.1016/0198-0149(91)90087-V Shirodkar P V, Mesquita A, Pradhan U K, et al. 2009. Factors controlling physico-chemical characteristics in the coastal waters off Mangalore—a multivariate approach. Environmental Research, 109(3): 245–257, doi: 10.1016/j.envres.2008.11.011 Smith S L. 1982. The northwestern Indian Ocean during the monsoons of 1979: distribution, abundance, and feeding of zooplankton. Deep-Sea Research Part A. Oceanographic Research Papers, 29(11): 1331–1353, doi: 10.1016/0198-0149(82)90012-7 Soetaert K, Van Rijswijk P. 1993. Spatial and temporal patterns of the zooplankton in the Westerschelde estuary. Marine Ecology Progress Series, 97: 47–59, doi: 10.3354/meps097047 Strickland J D H, Parsons T R. 1972. A Practical Handbook of Seawater Analysis. 2nd ed. Ottawa: Fisheries Research Board of Canada, doi: 10.25607/OBP-1791 Subrahmanya K R, Jayappa K S. 1987. Origin of netravati-gurupur estuarine system. Proc Natn Sem. Estuarine Management, Trivandrum: 70–72 Sulochanan B, Dineshbabu A P, Saravanan R, et al. 2014. Occurrence of Noctiluca scintillans bloom off Mangalore in the Arabian Sea. Indian Journal of Fisheries, 61(1): 42–48 Sun D, Liu Z S, Wang C S. 2016. Scale-dependent environmental control of mesozooplankton community structure in three aquaculture subtropical bays of China. Oceanologia, 58(2): 124–134, doi: 10.1016/j.oceano.2015.11.002 Tokioka T. 1979. Neritic and oceanic plankton. In: van der Spoel S, s Pierrot-Bults A C, eds. Zoogeography and Diversity of Plankton. Utrecht: Bunge Science, 126–143 Turner J T. 1984. Zooplankton feeding ecology: contents of fecal pellets of the copepods Temora turbinata and T. stylifera from continental shelf and slope waters near the mouth of the Mississippi River. Marine Biology, 82(1): 73–83, doi: 10.1007/BF00392765 Turner J T. 1986. Zooplankton feeding ecology: contents of fecal pellets of the cyclopoid copepods Oncaea venusta, Corycaeus amazonicus, Oithona plumifera, and O. simplex from the northern Gulf of Mexico. Marine Ecology, 7(4): 289–302, doi: 10.1111/j.1439-0485.1986.tb00165.x Turner J T. 2004. The importance of small planktonic copepods and their roles in pelagic marine food webs. Zoological Studies, 43(2): 255–266 Uye S I. 1994. Replacement of large copepods by small ones with eutrophication of embayments: cause and consequence. Hydrobiologia, 292(1): 513–519, doi: 10.1007/BF00229979 Vannucci M, Santhakumari V, dos Santos E P. 1970. The ecology of hydromedusae from the Cochin area. Marine Biology, 7(1): 49–58, doi: 10.1007/BF00346808 Venkataramana V, Kerkar A U, Mishra R K, et al. 2022. Hydrodynamics and zooplankton assemblages in Prydz Bay, East Antarctica, during the austral summer of 2017. Regional Studies in Marine Science, 52: 102341, doi: 10.1016/j.rsma.2022.102341 Venkataramana V, Sarma V V S S, Matta Reddy A. 2017. River discharge as a major driving force on spatial and temporal variations in zooplankton biomass and community structure in the Godavari estuary India. Environmental Monitoring and Assessment, 189(9): 474, doi: 10.1007/s10661-017-6170-5 Vineetha G, Kripa V, Karati K K, et al. 2022. Surge in the jellyfish population of a tropical monsoonal estuary: a boon or bane to its plankton community dynamics?. Marine Pollution Bulletin, 182: 113951, doi: 10.1016/j.marpolbul.2022.113951 Vineetha G, Madhu N V, Kusum K K, et al. 2015. Seasonal dynamics of the copepod community in a tropical monsoonal estuary and the role of sex ratio in their abundance pattern. Zoological Studies, 54(1): 54, doi: 10.1186/s40555-015-0131-x Vivekanandan E. 2005. Stock Assessment of Tropical Marine Fishes. New Delhi: Indian Council of Agricultural Research Waggett R, Costello J H. 1999. Capture mechanisms used by the lobate ctenophore, Mnemiopsis leidyi, preying on the copepod Acartia tonsa. Journal of Plankton Research, 21(11): 2037–2052, doi: 10.1093/plankt/21.11.2037 Wellershaus S. 1969. On the taxonomy of planctonic Copepoda in the Cochin Backwater (a South Indian Estuary). Bremen: Kommissionsverlag F. Leuwer Wickstead J H. 1962. Food and feeding in pelagic copepods. Proceedings of the Zoological Society of London, 139(4): 545–555, doi: 10.1111/j.1469-7998.1962.tb01593.x Yang Guanming, He Dehua, Wang Chunsheng, et al. 1999. Study on the biological oceanography characteristics of planktonic copepods in the waters north of Taiwan Island II. Community characteristics. Haiyang Xuebao (in Chinese), 21(2): 72–80 -
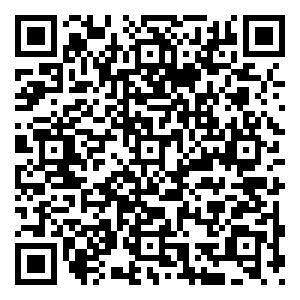
计量
- 文章访问数: 104
- HTML全文浏览量: 39
- 被引次数: 0