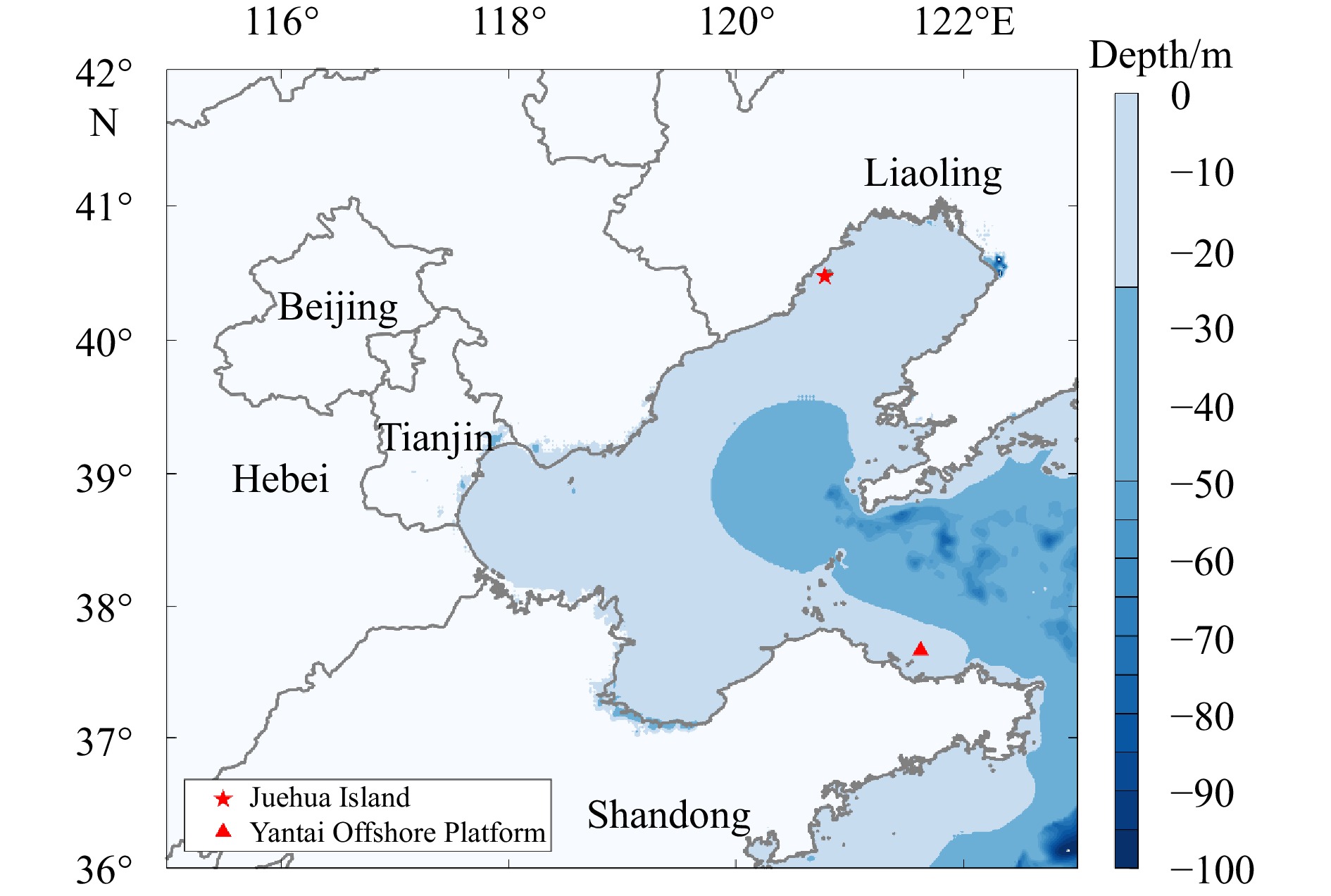
Citation: | Tan Yu, Yuhan Xia, Zhengli Qiu, Bangyi Tao, Yan Bai, Xianqiang He, Bing Chen, Mingxing Li, Yu Wang, Qilan Zhang, Chao Liang. Field observation of air-sea CO2 and H2O flux using the eddy covariance method based on 100 Hz gas analyzer in the Bohai and Yellow Seas[J]. Acta Oceanologica Sinica. doi: 10.1007/s13131-024-2393-9 |
CO2 is the most important anthropogenic greenhouse gas, and its increasing concentration is accelerating climate change (Liu et al., 2022; IPCC, 2023). Furthermore, water vapor is also an important greenhouse gas (GHG), and changes in its tropospheric concentration affect climate while influencing the concentration of other GHGs in the atmosphere (Wuebbles et al., 1989; Coe et al., 2021). Accurate estimates of air-sea CO2 and water vapor fluxes are crucial to accurately predict and respond to climate change (Hart, 2006; Brevik, 2012; Kaye and Quemada, 2017; Shukla et al., 2019).
In situ observation, using either a box method or a micrometeorological method, is an important method for studying air-sea flux (Hu et al., 2014). For different downwelling environmental conditions and the needs of long-term and continuous in situ observation, the micrometeorological method (based on the principles of atmospheric thermodynamics and dynamics) is the best choice for air-sea flux observation (Siebicke and Emad, 2019). Micrometeorological methods include eddy covariance (EC) methods, mass balance methods, and vorticity accumulation methods (Baldocchi, 2003; Baldocchi et al., 1988; Mammarella et al., 2009; Palatella et al., 2014; Weber, 1999). The EC, which is a direct air-sea flux measurement technique with the advantages of requiring no empirical parameters and being easy to use for in situ observation, has undergone substantial development in recent decades (Horst, 2000; Eugster et al., 2000; Baldocchi, 2003; Shahan et al., 2022).
The eddy correlation method was originally proposed by Swinbank (1951) for the measurement of land-air heat, mass, and momentum changes. With the development of equipment (anemometers, computer storage media) and theory (hydrodynamics, micrometeorology), the method became more widely used (Matthews and Schume, 2022; Shahan et al., 2022; Czubaszek and Wysocka-Czubaszek, 2023; Rytwo and Eliyahou, 2023; Stagakis et al., 2023). As wobble platform calibration methods continue to be refined, there has been an increase in the number of studies focusing on air-sea flux observations carried out on instrumented platforms such as ships, buoys, and offshore platforms (Horst, 2000; Kondo and Tsukamoto, 2007; Miller et al., 2008, 2010; Else et al., 2011; Blomquist et al., 2012).
The rest of this paper is organized as follows. The study area and data, including the experimental platform and instrumentation setup are described in Section 2; the basic principles of the EC, quality control, and quality assessment methods are presented in Section 3; the results of the calibration, quality assessment, and air-sea flux calculations of the high-frequency and low-frequency data are analyzed and discussed in Section 4; finally, conclusions and perspectives are presented in Section 5.
In this study, air-sea CO2 and water vapor flux observation experiments were carried out at the Yantai National Satellite Ocean Calibration Platform (hereafter referred to as the offshore platform) and the Sea Island Observation Platform (hereafter referred to as the sea island) at the Juehua Island Monolithic Beach Pier (Fig. 1). The offshore platform is 20 km away from land, which is conducive to the use of the eddy correlation method for air-sea interface flux observation. The island observation site is between the ocean and the land, which is conducive to understanding offshore air-sea exchange.
In current research on air-sea flux, few scholars have obtained such extensive field observation data for analysis. Many studies often choose to use near-shore meteorological observation stations or coastal scanning LiDAR (sLiDAR) for flux measurements, but both of our two observation platforms are island-based, allowing for direct measurements using instruments. By using a large number of direct sea observation data for subsequent air-sea flux calculations and comparative analysis between high and low frequency instruments, the results promise more accuracy, reliability, and significance for reference.
The offshore platform was fitted with a LI-
The Licor observation data storage cycle is 4 h. Instrument failure will lead to the loss of the entire 4 h data. High-frequency pulsometer data acquisition and storage can be accomplished at the same time by connecting the instrument to the host computer through the data collector network port for data viewing and export, to facilitate the debugging of the instrument, and prevent data loss.
Only high-frequency observations of water vapor were made on the island. Fig. 3b shows the water vapor field observation platform on Island, which carries an ultrasonic anemometer, a high-frequency water vapor pulsometer, and a Licor analyzer, as shown in Fig. 3d. Instruments were set up at a height of 2 m above the ground at a distance of about 10 m from the shore, which was also necessary to avoid being blocked by surrounding obstacles as much as possible.
The air-sea flux observations were carried out at the offshore platform and the island in August 2020 and July 2021, respectively. The observations contained three-dimensional wind speed (
Instrument /parameter |
Wind speed range | Wind speed accuracy | Wind direction range | Wind accuracy | Measuring frequency | Installation site |
HS-100 | 0-45 m/s | <1.0% RMS | 0-359° | <±1.0°RMS | 100 Hz | Island & Platform |
Instrument/parameter | Concentration | Measuring frequency | Installation site |
Licor-7500A | 0—50 mmol/mol 0—3 000 10–6 |
10 Hz | Island |
Licor-7500DS | 0—50 mmol/mol 0—3 000 10–6 |
20 Hz | Platform |
*CO2/H2O High frequency pulsometer | * | 100 Hz | Island & platform |
Note: The symbol “*” indicates that the measuring range is limited by reference to the Licor measuring range. |
Temperature and pressure data were used to facilitate air-sea flux calculations. The units of CO2 and H2O concentrations were converted from volume concentration to bulk density uniformly using the following conversion formula:
$$ \rho =\frac{cPM}{R(T+273.15)} , $$ | (1) |
where
The eddy covariance method uses fast response sensors to directly measure state variables such as wind speed, temperature, humidity, and carbon dioxide concentration. The covariance of the relevant physical quantities—such as water vapor and carbon dioxide density, with the values of vertically oriented wind speed pulsations (Baldocchi, 2003; Landwehr et al., 2018)—is used to obtain the air-sea fluxes of water vapor and carbon dioxide:
$$ F=\overline{{w}{{\prime}}{\rho }{{\prime}}} , $$ | (2) |
where
The first step in air-sea flux calculation using eddy covariance methods involves a series correction of the raw data. Since the high and low-frequency observations are based on the TDLAS and NDIR techniques, respectively, there are some systematic errors in the observations of both. We first performed a systematic error correction on the TDLAS high-frequency pulsometer using the Licor gas analyzer results as a benchmark. The data processing flow after the error correction is shown in Fig. 4. Importantly, the WPL correction compensates for expected physical variability due to temperature and water (Burba, 2013), and the instrumental or measurement errors are not considered. Therefore, only the WPL corrected air-sea fluxes are discussed in Section 4.1.4 of this paper.
To minimize the measurement error as much as possible as well as to ensure the integrity of the data information, the measurement noise in this study was preprocessed using the measurement range limitation, outlier rejection of the Pauta criterion, stiff value test, and random pulse rejection. The specific steps are as follows.
(1) Measurement range limitation
According to the measurement range of the instrument, the values beyond the measurement range of the instrument were rejected, as shown in Tables 1 and 2.
(2) Pauta criterion to eliminate outliers
We used the Pauta criterion to identify values outside the range of
(3) Stiff value test
During the measurement process, the ultrasound probe and the data acquisition system can have consecutive and extremely small changes in data values within a certain period, which is because the difference between neighboring points is less than a certain threshold value. For example, for a time series
(4) Random pulse rejection
Random electrical signal pulses in the observation equipment and data acquisition system can lead to random pulses in the data. Random pulse screening was performed as follows: first, a moving window of length 100 was used to calculate its standard deviation; when a data point exceeded the standard deviation by a factor of 3.5 it was regarded as a random pulse and replaced using linear interpolation between data points. Next, the window was moved so that when four or more consecutive points were detected as random pulses, they were not considered as such (Højstrup, 1993; Vickers and Mahrt, 1997).
Factors such as changing meteorological conditions and sensor drift can affect data changes. Usually, a systematic increase or decrease in data is considered a linear trend (Rannik and Vesala, 1999). To eliminate such systematic variations, we applied a linear detrending method to the data.
There are
$$ {\bar{c}}_{k}=\bar{c}+b\left({t}_{k}-\frac{1}{N}{\sum }_{k=1}^{N}{t}_{k}\right) , $$ | (3) |
where
$$ b=\frac{\displaystyle{\sum }_{k=1}^{N}{c}_{k}{t}_{k}-\frac{1}{N}{\sum }_{k=1}^{N}{c}_{k}{\sum }_{k=1}^{N}{t}_{k}}{\displaystyle{\sum }_{k=1}^{N}{t}_{k}{t}_{k}-\frac{1}{N}{\sum }_{k=1}^{N}{t}_{k}{\sum }_{k=1}^{N}{t}_{k}} , $$ | (4) |
The trend value
$$ {c}_{{k}_{d}}={c}_{k}-{\bar{c}}_{k} . $$ | (5) |
Open-circuit sensors with a certain processing time delay lead to a certain fixed time delay that causes the overall observed data to be skewed (Mauder and Foken, 2015). The time delay between the ultrasonic anemometer and the gas analyzer is determined by the maximum correlation value between the CO2/H2O concentration and
$$ CC\left(c,w,t\right)=\frac{\displaystyle\frac{1}{N}{\sum }_{i=1}^{N}{c}{\prime}\left(i\right){w}{\prime}\left(i+t\right)}{{\sigma }_{c}{\sigma }_{w}} , $$ | (6) |
$$ {c}{\prime}=c\left(i\right)-\frac{1}{N}{ \displaystyle\text{Σ} }_{j=1}^{N}c\left(j\right) , $$ | (7) |
$$ {w}{\prime}=w\left(i\right)-\frac{1}{N}{ \text{Σ} }_{j=1}^{N}w\left(j\right) , $$ | (8) |
$$ {\sigma }_{c}=\sqrt{\frac{1}{N}{\sum }_{i=1}^{N}{c}{{'}2}} , $$ | (9) |
$$ {\sigma }_{w}=\sqrt{\frac{1}{N}{\sum }_{i=1}^{N}{w}{{'}2}} . $$ | (10) |
where
The basic condition for the eddy correlation method is that the vertical mean wind speed is zero. For a flat subsurface, the wind speed
In this paper, the measured wind speed data were corrected using the DR method (Geernaert, 1988; Wilczak et al., 2001).
First, the first rotation is carried out so that
$$ \left\{\begin{array}{c}{u}_{1}=u\mathit{cos}\theta +v\text{sin}\theta \\ {v}_{1}=-u\text{sin}\theta +v\text{cos}\theta \\ {w}_{1}=w\end{array}\right. , $$ | (11) |
where
Next, a second rotation is carried out so that
$$ \left\{\begin{array}{c}{u}_{2}={u}_{1}\text{cos}\phi +{w}_{1}\text{sin}\phi \\ {v}_{2}={v}_{1}\\ {w}_{2}=-{u}_{1}\text{sin}\phi +{w}_{1}\text{cos}\phi \end{array}\right. , $$ | (12) |
where
To compare the effect of different observation frequencies on the air-sea flux, the observations were time-matched. The mean value of the sampled values less than 1/2 of the matched time resolution was the matched point value. The points that were not matched to a value were supplemented with NaN values. Taking the 20 Hz standard time grid as an example, the center moment of its grid is
The WPL correction is used to correct for fluctuations in CO2 and H2O densities due to water vapor and temperature fluctuations in open-optical path gas analyzers (Jentzsch et al., 2021).
$$ {F}_{c}=\overline{{w}{\prime}{\rho }_{c}{\prime}}+\mu \frac{\overline{{\rho }_{c}}}{\overline{{\rho }_{a}}}\overline{{w}{\prime}{\rho }_{w}{\prime}}+\left(1+\mu \sigma \right){\bar{\rho }}_{c}\frac{\overline{{w}{\prime}{T}{\prime}}}{\overline{T}} , $$ | (13) |
$$ {F}_{w}=\left(1+\mu \sigma \right)\left(\overline{{w}{\prime}{\rho }_{w}{\prime}}+\overline{{\rho }_{w}}\frac{\overline{{w}{\prime{T}{\prime}}}}{\overline{T}}\right) , $$ | (14) |
where
In this section, the observed data were subjected to turbulence spectral analysis, a turbulence smoothness test, and a turbulence development adequacy test; the data quality was classified via Mauder’s grading criteria (Mauder and Foken, 2015) (Table 3).
Turbulence stability (%) | Turbulence development adequacy (%) | overall quality level |
<30 | <30 | 0 |
<100 | <100 | 1 |
>100 | >100 | 2 |
Note: * Level 0 is high quality data that can be used for basic research analysis; Grade 1 is medium quality data, which can be used for general air-sea flux analysis; Level 2 is low-quality data and should be discarded or interpolated. |
The one-dimensional wind speed vector conformed to the “Kolmogorov –5/3” law, i.e., the slope of the inertial subregion satisfied “–5/3” in the case of local uniform isotropy (Li et al., 2021a). To verify the form of energy transport in the entire system of atmospheric turbulence, the power spectrum and co-spectrum models proposed by Kaimal et al. (1972) based on the “Kolmogorov –5/3” law are commonly used. In other words, the power spectrum in the inertial subregion follows the “–2/3” law and the co-spectrum follows the “–4/3” law.
Turbulence spectral analysis is the power and co-spectral analysis of wind speed and water vapor or CO2 (Kaimal et al., 1972; Su et al., 2004):
$$ spectrapower:\frac{nS\left(n\right)}{{u}_{*}^{2}} , $$ | (15) |
$$ cospectra:\frac{nC{o}_{wc}\left(n\right)}{\overline{{w}^{\text{'}}{c}^{\text{'}}}} , $$ | (16) |
where
To ensure that the half-hourly turbulence statistics were stable, a turbulence smoothness test was performed using the following expression (Lee et al., 2005):
$$ \Delta st=\left|\frac{\overline{({w}{{'}}{c}{{'}}{)}_{5}}-\overline{({w}{{'}}{c}{{'}}{)}_{30}}}{\overline{({w}{{'}}{c}{{'}}{)}_{30}}}\right|\times 100\text{%} , $$ | (17) |
The subscripts “5” and “30” represent 5 and 30 min samples, respectively, in the same time period.
The adequacy of turbulence development can be expressed in terms of the overall turbulence characteristic coefficients (ITC, integral turbulence characteristics). Atmospheric turbulence is considered to be adequately developed if the ITC is < 30% (Kaimal et al., 1972; Lee et al., 2005). The ITC expression is:
$$ \text{IT}{\text{C}}_{\sigma }=\left|\frac{({\sigma }_{w}/{u}_{*}{)}_{\text{model}}-({\sigma }_{w}/{u}_{*}{)}_{\text{measured}}}{({\sigma }_{w}/{u}_{*}{)}_{\text{model}}}\right| , $$ | (18) |
where
$$ {\left(\frac{{\sigma }_{w}}{{u}_{*}}\right)}_{model}=\left\{\begin{array}{cc}1.3(1-3z/L{)}^{1/3}& z/L < -0.1\\ 1.4& -0.1 < z/L < 0.1\\ 1.5& z/L > 0.1\end{array}\right. , $$ | (19) |
where
The Obukhov length
$$ L=-\frac{{{u}_{*}}^{3}\theta }{kg\overline{{w}{\prime}{\theta }{\prime}}} , $$ | (20) |
where
In this study, data from the offshore platform in August 2020 and the island platform data in July 2021 were used as examples to analyze the air-sea flux observations at different frequencies. Three main aspects were included: data calibration, quality assessment, and differences in air-sea flux observed at different frequencies.
The maximum correlation coefficients of ultrasonic wind speed with water vapor and CO2 concentration were analyzed to determine the delay time using the observation data from the offshore platform, respectively (Fig. 5). The results show the delay times of the ultrasonic anemometer with the high-frequency water vapor pulsometer and the high-frequency CO2 pulsometer are −0.1 s and 1 s, respectively.
Figure 6 shows the changes in the 3-D wind speed vector before and after that DR that transforms the 3-D wind speed vector from the ultrasonic anemometer coordinate system to the natural coordinate system. The pre-correction image (a) shows that the vertical wind speed
The Ogive curve, which integrates the co-spectrum of the vertical wind speed
The Ogive curves of downwind and vertical wind speeds plotted for 16 randomly selected days from our July 2021 offshore platform observations, and randomly selecting 1 hour data each day. Each Ogive Curve corresponds to one hour of data from one of the days. We randomly select as much data as possible to ensure that the chosen data can represent the overall data quality. The results in Fig. 7 show that the Ogive value gradually increases over time. When the observation time reaches 1 min, the Ogive value gradually becomes stable; most of the data become completely stable at 5 min, and the Ogive value gradually approaches 1.0 m2/s2 at 30 min. Oncley et al. (1996) analyzed 10 Hz observation samples and found that the optimal observation length was between 5 min and 30 min, and the corresponding natural frequency was 3.3 × 10–3 Hz ~ 5.6 × 10–4 Hz. Unlike previous studies, the air-sea observation frequencies in this study were carried out at 100 Hz, which allowed for the detection of smaller and more frequent changes in turbulence. To analyze the influence of different observation frequencies on the air-sea flux, we examined the observed data of the air-sea fluxes from offshore platforms and islands, with the shortest duration (5 min) in Oncley et al. (1996).
Data in July 10, 2021 were selected as the island observations, which were more comprehensive than the other days, because it was more complete and allowed to analyze flux changes over the course of the day. As mentioned in Section 2.2, the island observations were only high-frequency observations of water vapor. Therefore, in this section, only the air-sea water vapor fluxes were corrected for WPL, and the changes in air-sea flux before and after the correction were analyzed.
Figure 8 shows that in terms of the magnitude of air-sea flux change, the WPL corrected water vapor flux was smaller than the uncorrected water vapor flux, which is consistent with the results of Jentzsch et al. (2021). The difference was that Fig. 8 has two corrected peaks at 09:00 and 19:25 due to the complex island observing environment. In terms of air-sea flux differences, the WPL correction has a significant effect on the calculated values of water vapor fluxes, with a maximum amplitude effect on air-sea fluxes of about 1.5 mmol/(m2·s). The air-sea flux differences before and after the correction varied with time and were significantly different. Between 0:00 and 9:00, the air-sea flux difference showed a clear upward trend; from about 9:00 to 18:10, the air-sea flux difference showed a downward trend accompanied by an increase in amplitude of about 1 h, which maintained a stable air-sea flux difference during the night. The results of the WPL corrections show that temperature and water vapor led to a change in the density of the observed amount, resulting in notable changes in air-sea flux change. The faster the temperature and water vapor changed, the larger the magnitude of the correction.
The wind speed power spectrum and its co-spectrum with CO2 and water vapor are analyzed from selected island observations. Fig. 9 shows that the inertial subregion was in the frequency range of approximately 1 Hz to 10 Hz. The peak value of the power spectrum appears at a frequency lower than 1 Hz, representing a region of high energy content known as the energy-containing vortex region, and the spectrum value in the range from 10–3 to 100 increases with the increase of frequency. When the frequency is about 1 Hz to 10 Hz, the power spectrum shows a downward trend, and the turbulent kinetic energy produces a turbulent cascade with isotropic characteristics. Parallel to the slope line, this part follows the −2/3 law, representing the inertial subregion. When the frequency is higher than 10 Hz, the spectral value change deviates from this slope line, which indicates the onset of the dissipation region. Here, turbulent kinetic energy is transferred to a smaller-scale vortex and dissipates into internal energy due to molecular viscosity.
In stable conditions (–0.1 < z/L≤ 1) at low frequencies, data from offshore platforms shows an upward trend as frequency decreases, while the island data shows a decreasing trend with the decrease of frequency. This opposite trend between the two instruments indicates that the 100 Hz instruments are more capable of capturing detailed changes in the low-frequency region.
Figure 10 shows the co-spectrum of vertical wind speed
The measured mean data were most consistent with the Kaimal et al. (1972) atmospheric instability (–1.0 < z/L ≤ –0.1) in the low-frequency region, and the trend of the scatter distribution in the high-frequency region was more consistent with the –4/3 slope. At frequencies less than 0.01 Hz, the co-spectral values were higher than those of Kaimal’s results due to factors such as insufficient sample data and noise. At frequencies higher than 10 Hz, small co-spectral densities may lead to larger measurement errors (Su et al., 2004). Combining the power spectrum and co-spectrum results, the high-frequency observations fulfil the power spectrum slope of –2/3 and the co-spectrum slope of –4/3 in the inertial sub-region.
Due to the short time series of the offshore platform observation data, which is not conducive to turbulence quality analysis, the island observation data were used to evaluate the overall turbulence quality of the air-sea fluxes and assess the overall quality of the water vapor flux data with three grades of 0, 1, and 2 (see Table 3).
Figure 11 shows the overall turbulence data quality after the turbulence smoothness test and the turbulence development adequacy test. The results show that high-quality data account for about 46.5% of the overall air-sea flux data, and 25.0% of the moderate-quality data. About 28.5% of the points to be discarded were distributed around 12:00 on July 10 and 11. Overall about 71.5% of the island water vapor flux data passed the turbulence smoothness test and the turbulence development adequacy test. In Fig. 11 around 14:00 on July 11, there is a data point with a value of 5 mmol/(m2·s) and QC = 1, which means that this is the general quality data, and around 20:00 in the evening of July 11, the water vapor flux has a value of –3 mmol/(m2·s) and QC = 2, which means that it is the low-quality data. It can be seen that the QC = 1 data interval used for the general quality analysis is quite scattered, with a large difference between the maximum and minimum values.
Figure 12 shows the air-sea flux observations from the offshore platform. The 100 Hz and 20 Hz air-sea flux trends were generally the same but with larger variations. Water vapor fluxes showed opposite trends for the high and low-frequency observations from 14:55 to 15:05 (Fig. 12a). This may be related to the fact that more turbulent changes are monitored by high-frequency observations, and it is normal for air-sea flux trends to be reversed on smaller timescale scales. At 16:45, the CO2 flux had a negative air-sea flux in high-frequency measurements and a positive flux in low-frequency measurements (Fig. 12b). These results indicate that the measurement frequency had an important effect on the air-sea flux, affecting both intensity and direction.
Figure 13a shows the difference between the high and low-frequency water vapor fluxes. These air-sea fluxes were small and less than 0.01 mmol/(m2·s) on July 9, while the difference between the fluxes on July 10 and 11 increased from about 0.005 to 0.03 mmol/(m2·s). According to the experimental record, there was rain on the island on July 10 and 11, which increased the water vapor density in the air and subsequently the air-sea fluxes. Figs. 13b, c, and d show the local magnification of the water vapor flux variations on July 9, 10, and 11, respectively. During this time, the water vapor fluxes varied between –0.2 and 1.00 mmol/(m2·s), with total air-sea fluxes being positive. From 15:21 to 15:43 on July 11, the air-sea flux peaks were lower in the high-frequency observations than in the low-frequency observations. An opposite trend was observed at 15:00 observation at the offshore platform (Fig. 12a). These two fluxes are not directly correlated and have different response rates for different concentrations in air, the source-sink variations in H2O and CO2 fluxes are not in one-to-one correspondence. During the field measurements, the weather and instrument shaking conditions were the same, and the performance was stable except for a sudden drop in the CO2 values at 15:00. This inconsistency in the sudden change may be due to the fact that the two fluxes were measured at some distance from each other in the field, which had a certain impact on the results. In addition to this, it can also be shown that this difference was caused by high-frequency observations that were able to capture additional information about turbulence changes, both upward and downward, on a different timescale. Taken together, the frequency of the observations had a smaller effect on the water vapor flux than on the CO2 flux, probably because the water vapor concentration in the atmosphere was much higher than the CO2 concentration.
Figure 14 shows the evolution over time of water vapor and CO2 concentrations. The high-frequency observations had more pulsed values and more pronounced amplitudes of change than the low-frequency observations. Observations from the offshore platform were smooth, with high water vapor concentrations and narrow variations. Long-term observations on the islands showed some cyclical variations in water vapor concentration, with a sudden drop in water vapor concentration on July 11. This systematic and abrupt change can be attributed to factors such as changes in meteorological conditions and sensor drift (Moncrieff et al., 2004). The change in the trend of the data from the offshore platforms was not as obvious. There is a downward trend in the data from the platforms on the islands. In terms of the overall performance of the data, the high-frequency observations were able to monitor more of the small changes in the turbulence than the low-frequency data.
Figure 15 comprehensively shows the variation of air-sea fluxes between the island and offshore platforms with wind speed. Despite higher overall wind speeds at the offshore compared to that of the offshore platform, their air-sea fluxes show opposite behaviors. One difference is that the air-sea flux varies in size; second, the trends in air-sea flux change differ. These air-sea fluxes are determined by two factors: wind speed and gas concentration. In the nearshore seas, the proximity to land promotes frequent gas exchange, resulting in a higher variability in air-sea flux values. The air-sea flux on the offshore platform decreases with increasing wind speed, while on the island, air-sea flux increases with the increase of wind speed. These results show that with higher wind speeds, the island environment has more vertical turbulence than the offshore platform, leading to increased atmospheric instability increases. As wind speed increases, the error in air-sea flux measurements also increases. There is no significant difference observed in measurement errors between high-frequency and low-frequency measurements. However, as wind speed increases, the difference in air-sea flux between the high and low-frequency measurements on the two platforms becomes more pronounced.
In Fig. 16, it is evident that water vapor fluxes from offshore platforms exhibit an inverse trend to CO2 fluxes, and opposite to the variation trend of water vapor flux on the islands. The water vapor flux of the offshore platform decreases with the increase in wind speed, and the water vapor flux value ranges from −0.1 mmol/(m2·s) to 0.2 mmol/(m2·s), while the wind speed ranges from 8 m/s to 11 m/s, indicating low and intermediate wind speeds. The ambient water vapor flux ranges from −0.2 mmol/(m2·s) to 1 mmol/(m2·s), and the wind speed ranges from 0 m/s to 8 m/s. The overall water vapor flux direction of the island environment trends upward, while the water vapor flux of the offshore platform is balanced and the water vapor exchange is weak. For CO2 flux, the overall air-sea flux direction trends upward, and the ocean is the source of CO2 release. In obtaining this result, we did not take into account the problem of the distance from the installation position of the instrument, and we hope to further improve the accuracy of our results in subsequent experiments. In terms of basic variation trends and errors, the results in Fig. 16 are consistent with those in Fig.15.
The above results show that the trends of variation in air-sea flux with wind speeds are notably different due to differences in the underlying surface environment and atmospheric stability. With the increase of wind speed, the air-sea flux measurement error increases, so the uncertainty of observation frequency to air-sea flux increases. However, the overall difference between 100 Hz and 20 Hz errors is not large, and the observed air-sea flux values are different.
There are few reports on gas analyzers at 100 Hz, and there is no fixed relationship between air-sea CO2 and water vapor flux and wind speed. This is because wind speed mainly changes the transfer velocity without altering the magnitude of the partial pressures of CO2 or water vapor. The carbon source or sink primarily depends on the difference in CO2 partial pressure between the atmosphere and seawater. If the atmospheric CO2 partial pressure is greater, the ocean acts as a carbon sink, and if the ocean's CO2 partial pressure is greater, the ocean acts as a carbon source. The difference in CO2 partial pressure varies across different regions, hence the relationship between flux and wind speed will differ. This paper mainly aims to illustrate the differences in air-sea CO2 and water vapor flux observed at 20 Hz and 100 Hz, as well as the fact that high-frequency observations are superior to low-frequency observations under high wind speed conditions, through Fig. 16. The work of Li et al. (2021b) can support our conclusion.
Changes in turbulence occur very quickly, and thus concentrations and densities change accordingly. It is therefore necessary to use instruments with high accuracy and fast measurement data transfer rates, especially in windy environments. (1) Due to the limitation of low frequency of observation instruments, it often happens that the inertial domain band of the measured spectrum is too narrow to fit the Kolmogorov-5/3 power law well. The turbulence energy spectrum obtained from high-frequency observation covers not only the inertial subregion, but also part of the viscous subregion, which is conducive to calculating the turbulence parameters more accurately, and to identifying and analyzing turbulence characteristics. (2) When using Ogive Curves to calculate the averaging time, low-frequency observations may mask or smooth out some transient features of turbulence, while high-frequency observations can reduce this effect and make the observations closer to the real situation. Also, due to the intermittent nature of turbulence, turbulent and non-turbulent layers that are clearly bounded in low-frequency observations may underestimate certain fluxes. High-frequency observations can reduce this underestimation by capturing more turbulent activity, estimating turbulent fluxes more accurately, and tracking transient changes in turbulence. In addition to this, high-frequency observations provide data with higher temporal resolution, which page means that rapid changes in turbulence over time can be observed in more detail.
In this paper, we used water vapor and CO2 flux observations from offshore platforms and island platforms to carry out comprehensive data calibration and data quality, focusing on the effects of 100 and 20 Hz observing frequencies on air-sea flux observations. Different durations between different frequencies were found to have a great influence on the air-sea flux calculations. We chose a duration of 5 min for these calculations. Based on the results of the turbulence spectrum analysis, turbulence smoothness, and turbulence development adequacy tests, the observed data met the requirements for accurate air-sea flux calculations. The temperature and water vapor density corrections reduced the air-sea flux magnitude to some extent, and further reduced the air-sea flux differences caused by different observation frequencies. At low wind speeds, there were more high-frequency observations with different air-sea flux magnitudes and directions than low-frequency observations. The frequency of the wind speed observations was not the main factor contributing to the air-sea flux differences; rather, it was the magnitude of wind speed that contributed to the differences between high and low-frequency observations. At high wind speeds, the difference between high and low-frequency water vapor fluxes was small, and there was an overall large difference in CO2 fluxes. The results regarding the effects of environmental factors such as wind speed, temperature, and water vapor on the air-sea fluxes under high-frequency and low-frequency observations showed that high-frequency observations could provide more detailed information on turbulence variations than low-frequency data. Furthermore, changes in the air-sea fluxes of gases with lower concentrations in the atmosphere could be observed on short time scales.
Instrument /parameter |
Wind speed range | Wind speed accuracy | Wind direction range | Wind accuracy | Measuring frequency | Installation site |
HS-100 | 0-45 m/s | <1.0% RMS | 0-359° | <±1.0°RMS | 100 Hz | Island & Platform |
Instrument/parameter | Concentration | Measuring frequency | Installation site |
Licor-7500A | 0—50 mmol/mol 0—3 000 10–6 |
10 Hz | Island |
Licor-7500DS | 0—50 mmol/mol 0—3 000 10–6 |
20 Hz | Platform |
*CO2/H2O High frequency pulsometer | * | 100 Hz | Island & platform |
Note: The symbol “*” indicates that the measuring range is limited by reference to the Licor measuring range. |
Turbulence stability (%) | Turbulence development adequacy (%) | overall quality level |
<30 | <30 | 0 |
<100 | <100 | 1 |
>100 | >100 | 2 |
Note: * Level 0 is high quality data that can be used for basic research analysis; Grade 1 is medium quality data, which can be used for general air-sea flux analysis; Level 2 is low-quality data and should be discarded or interpolated. |
Instrument /parameter |
Wind speed range | Wind speed accuracy | Wind direction range | Wind accuracy | Measuring frequency | Installation site |
HS-100 | 0-45 m/s | <1.0% RMS | 0-359° | <±1.0°RMS | 100 Hz | Island & Platform |
Instrument/parameter | Concentration | Measuring frequency | Installation site |
Licor-7500A | 0—50 mmol/mol 0—3 000 10–6 |
10 Hz | Island |
Licor-7500DS | 0—50 mmol/mol 0—3 000 10–6 |
20 Hz | Platform |
*CO2/H2O High frequency pulsometer | * | 100 Hz | Island & platform |
Note: The symbol “*” indicates that the measuring range is limited by reference to the Licor measuring range. |
Turbulence stability (%) | Turbulence development adequacy (%) | overall quality level |
<30 | <30 | 0 |
<100 | <100 | 1 |
>100 | >100 | 2 |
Note: * Level 0 is high quality data that can be used for basic research analysis; Grade 1 is medium quality data, which can be used for general air-sea flux analysis; Level 2 is low-quality data and should be discarded or interpolated. |